编辑推荐: |
本文主要介绍了自动驾驶数据闭环相关内容。
希望对你的学习有帮助。
本文来自于CSDN,由火龙果软件Linda编辑,推荐。 |
|
最近自动驾驶和数据闭环结合在一起成为一大解决方案,原因是自动驾驶工程已经被认可是一个解决数据分布“长尾问题”的任务,时而出现的corner
case(极端情况)是对数据驱动的算法模型进行升级的来源之一,如图所示。
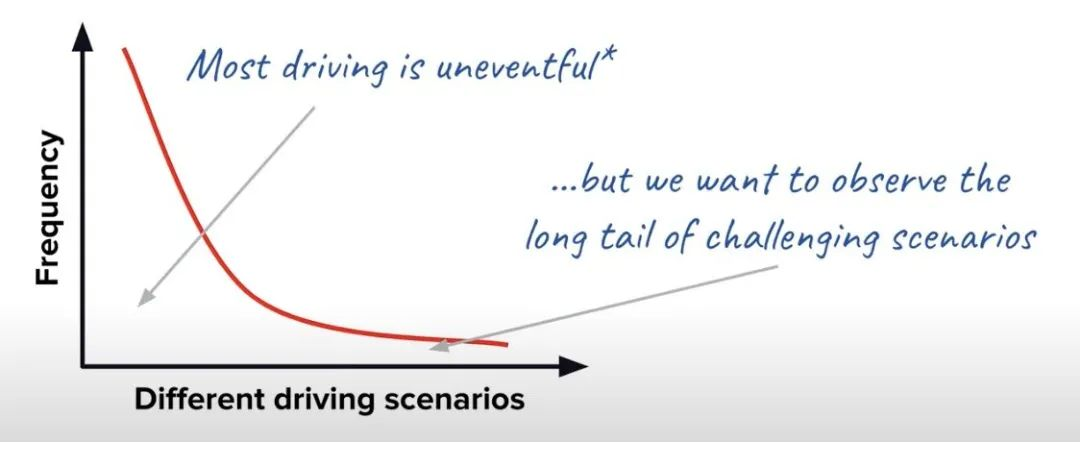
构成这个自动驾驶数据闭环的核心技术和模块都有哪些呢?首先是这个自动驾驶的算法和模块是数据驱动的,其次源源不断的数据需要有合理有效的方法去利用。
如图是Tesla众所周知的Autopilot数据引擎框架:确认模型误差、数据标注和清洗、模型训练和重新部署。
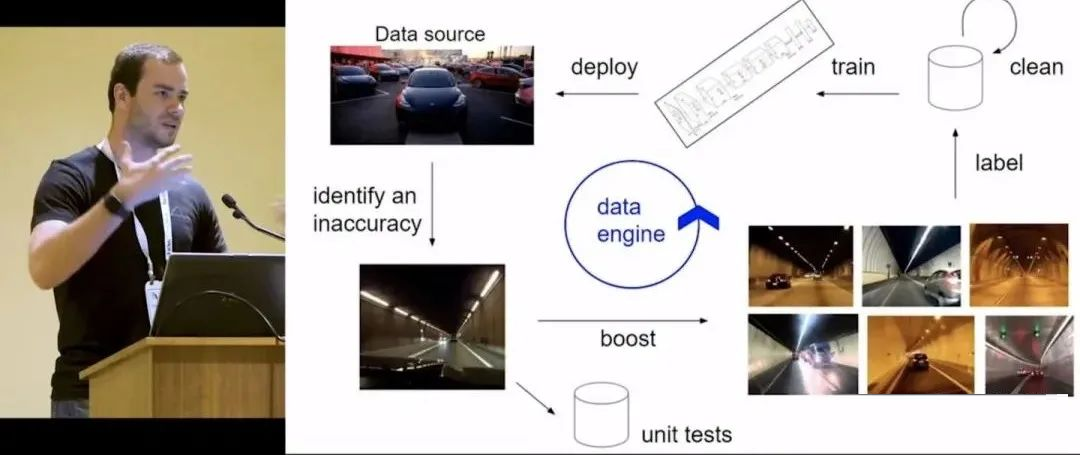
这是谷歌waymo报告提到的数据闭环平台:其中有数据挖掘、主动学习、自动标注、自动化模型调试优化、测试校验和部署发布。
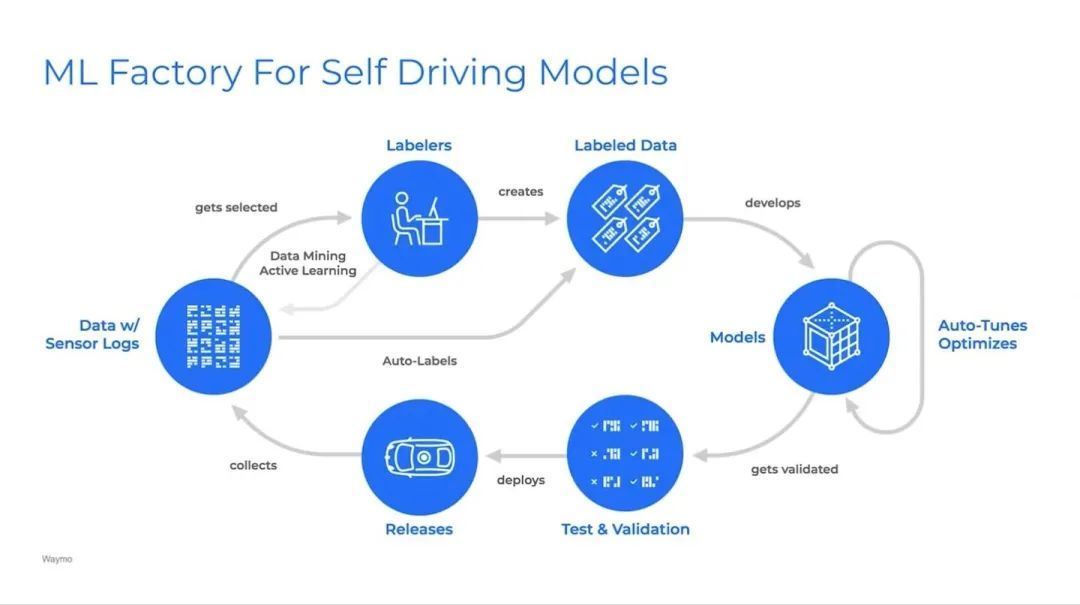
如图是英伟达公司在自动驾驶开发建立的机器学习平台MAGLEV,也是基于闭环的模型迭代:其中有smart的数据选择、数据标注、模型搜索、训练、评估、调试和部署。
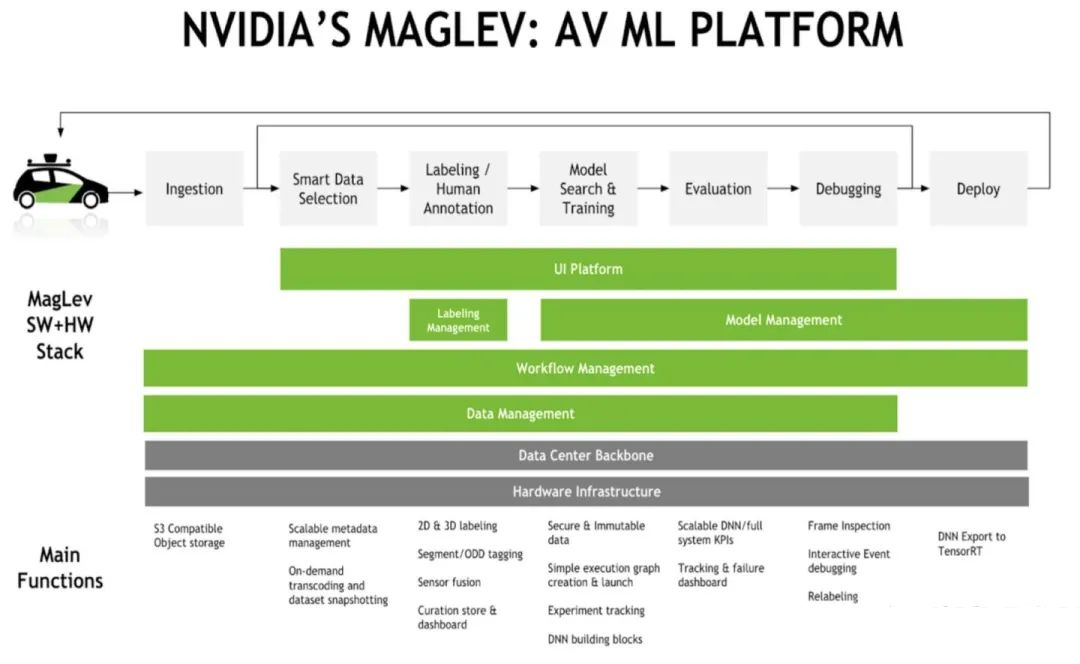
下面对数据闭环各个组成部分进一步讨论:
自动驾驶的数据驱动模型;
云计算平台的基建和大数据处理技术;
训练数据标注工具;
大型模型训练平台;
模型测试和检验;
相关的机器学习技术。
1 自动驾驶的数据驱动模型
应该说,自动驾驶的算法模块,基本都是数据驱动的训练模型要优于基于规则或者优化的,尤其是感知和预测。
以下图(综述论文 “A Survey of Autonomous Driving: Common
Practices and Emerging Technologies”)为例,现在自动驾驶的开发基本是模块化的(a),只有个别是采用端到端模式(b)。
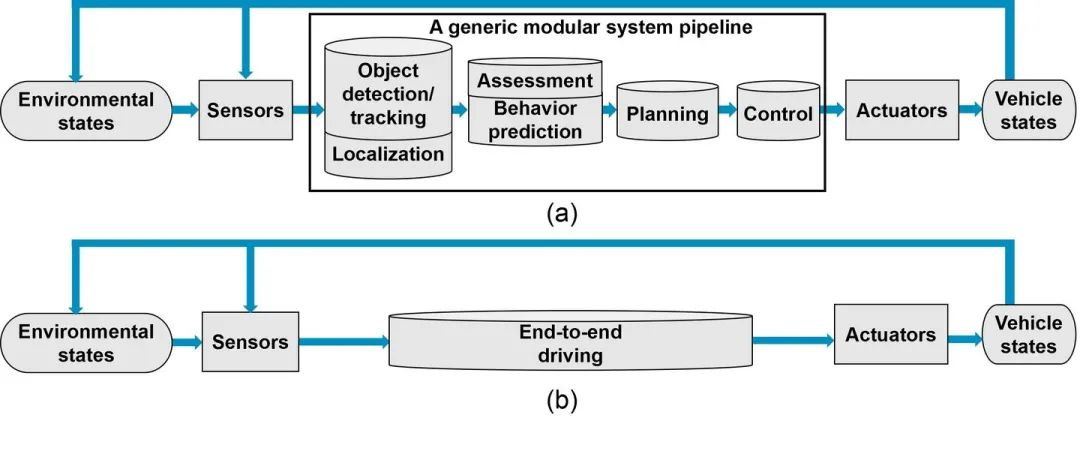
端到端模式可以说绝对是数据驱动的(如图为例),因为传统的优化和规则方法无法处理如此复杂的系统设计和公式化。
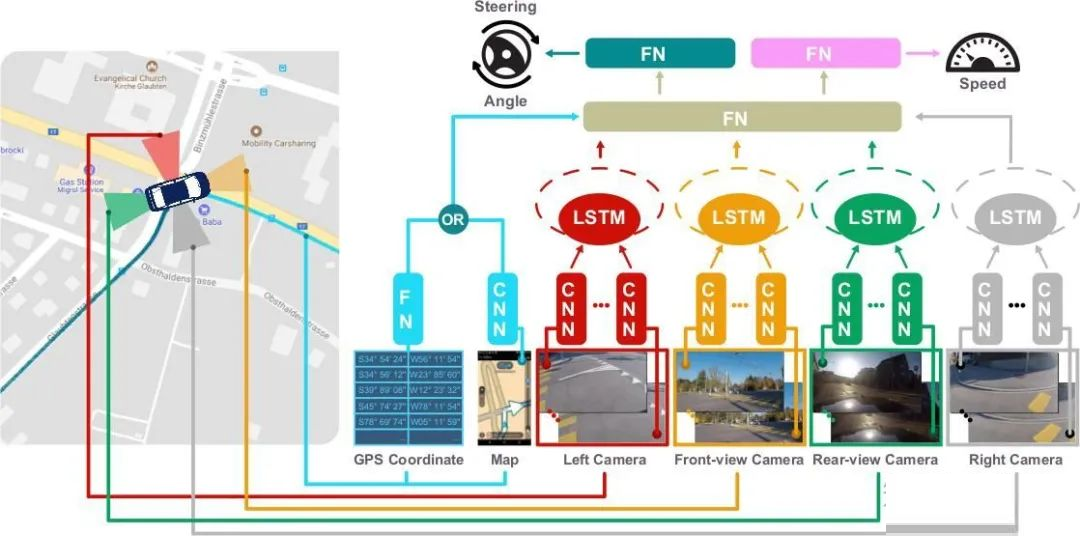
“E2E Learning of Driving Models with Surround-View
Cameras and Route Planners”
模块化的方法也是可以采用数据驱动方式,可以分成以下模块:
感知:图像/激光雷达/毫米波雷达
地图+定位
预测(感知-预测)
规划决策(预测-规划)
控制(规划-控制)
传感器预处理
模拟仿真
下面以此分别讨论:
1)感知:2-D/3-D 目标检测和分割基本是采用深度学习模型,无论激光雷达、摄像头或者传感器融合的形式;跟踪基本是tracking-by-detection方式,不过把跟踪和检测集成在一起做深度学习模型也是大家讨论的热点之一。
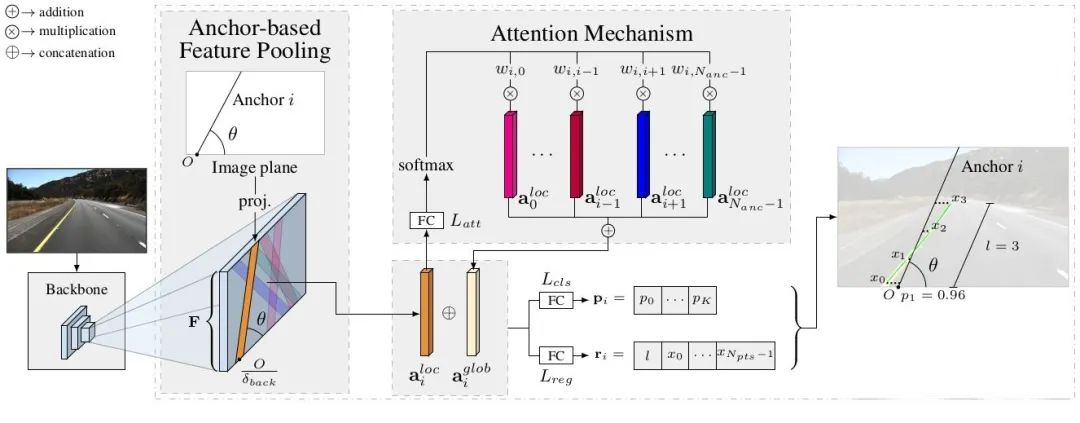
“Keep your Eyes on the Lane: Real-time Attention-guided
Lane Detection”
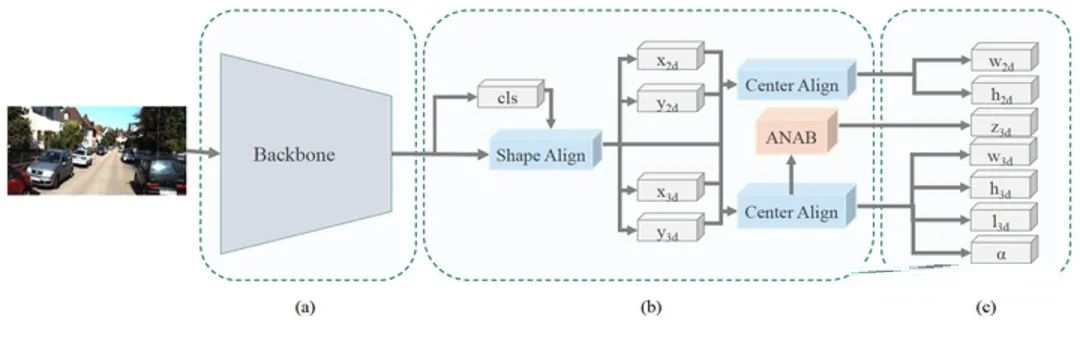
“M3DSSD: Monocular 3D Single Stage Object Detector”
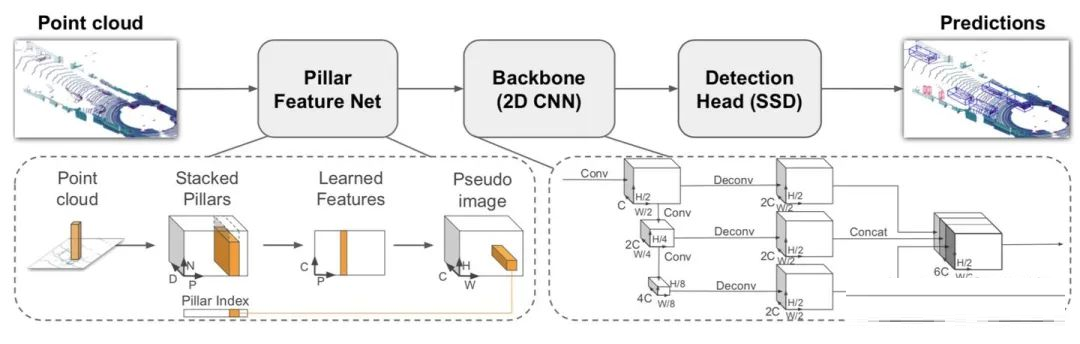
“PointPillars: Fast Encoders for Object Detection
from Point Clouds”
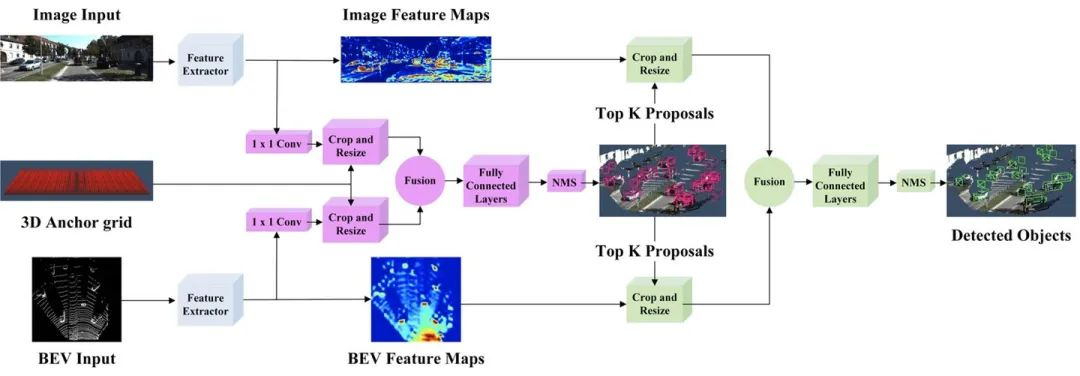
“Joint 3D Proposal Generation and Object Detection
from View Aggregation”
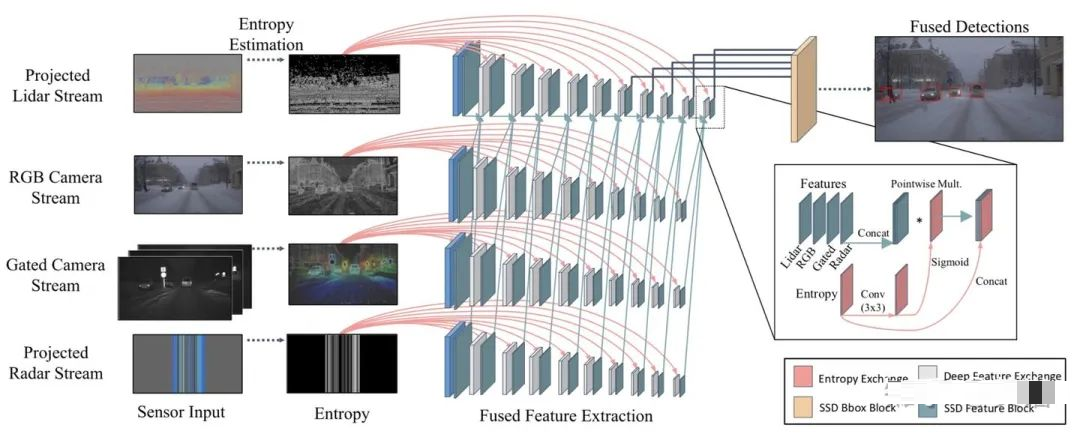
”Seeing Through Fog: Deep Multimodal Sensor Fusion
in Unseen Adverse Weather“
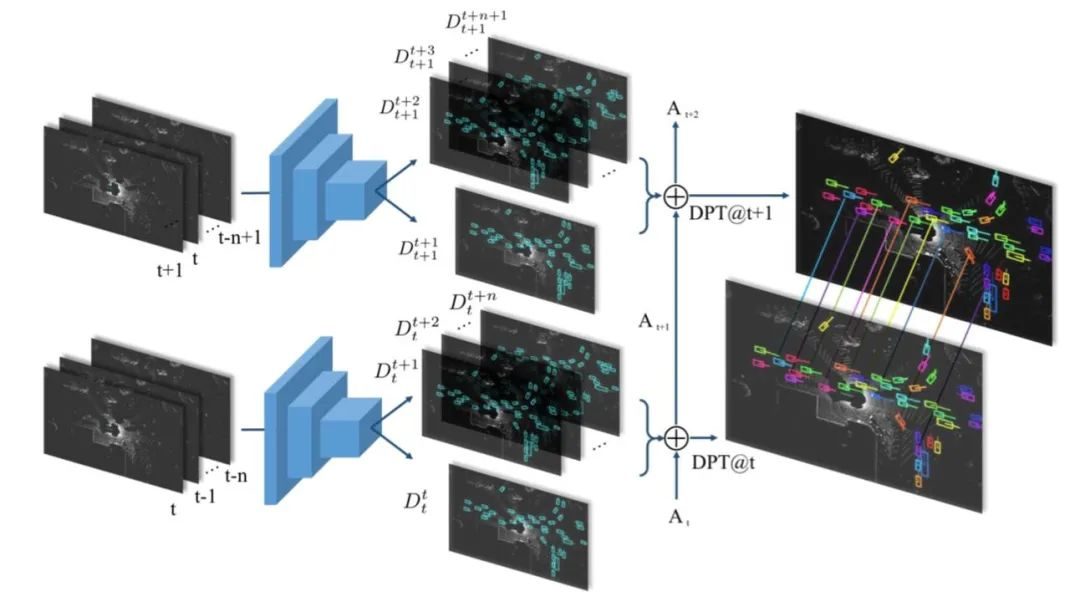
“Fast and Furious: R-T E2E 3D Detection,
2)地图定位:车辆定位中基于语义地图的方法,在语义目标提取也是采用深度学习模型,甚至语义地图的制作也是如此,目前感兴趣的地图更新(或者在线地图)多半需要检测语义目标。定位的后端也可以是数据驱动的解决,包括全局定位和相对定位,最近SLAM和深度学习的结合工作也如火如荼进行。
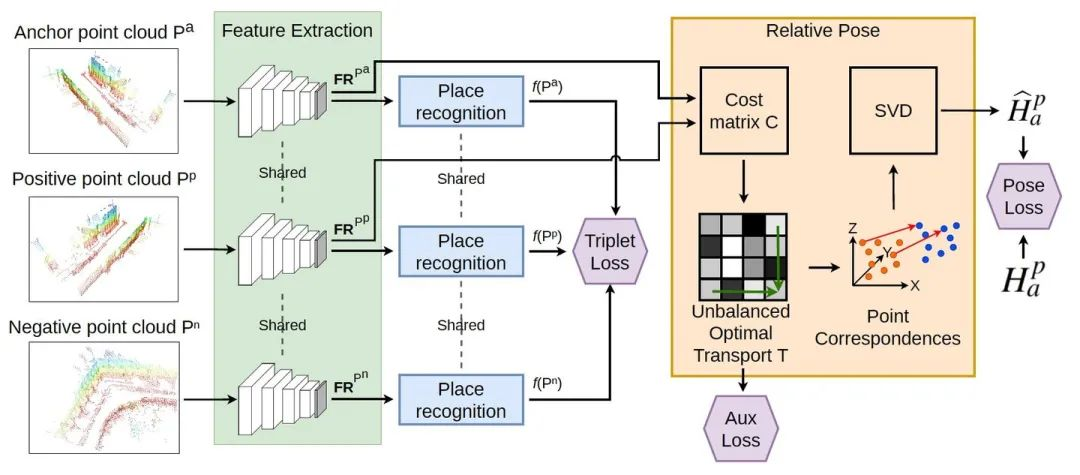
“LCDNet: Deep Loop Closure Detection andPoint Cloud
Registration for LiDAR SLAM”
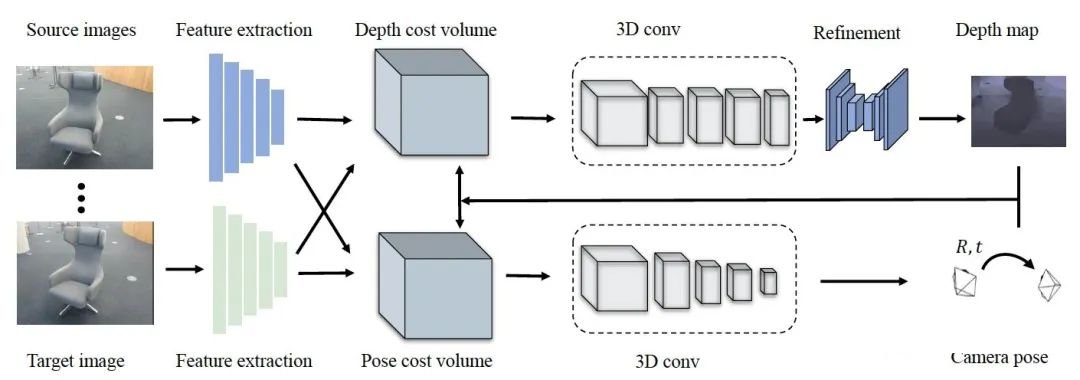
“DeepSFM: Structure From Motion Via DeepBundle Adjustment”
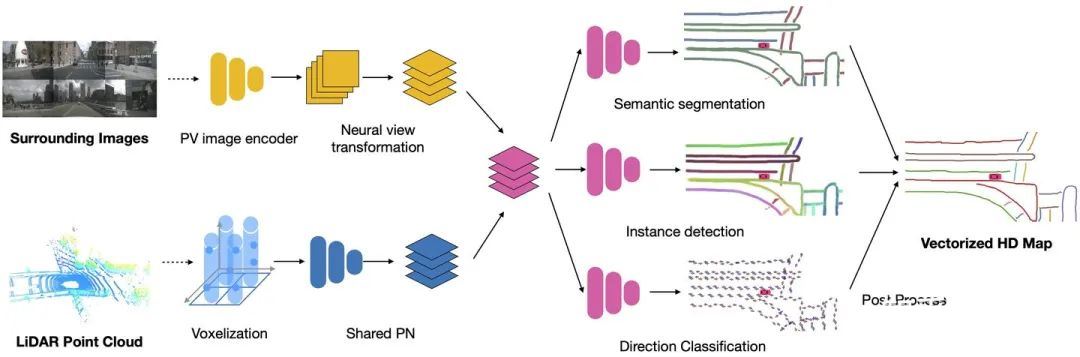
“HDMapNet: An Online HD Map Construction and Evaluation
Framework”
3)预测:障碍物的轨迹预测现在已经是自动驾驶发展至今的重点之一,现在重要性可以说是高于感知。如何建模智体行为、如何建模智体之间的交互和轨迹预测的动作多模态性,自然最佳方式是采用数据来训练。另外,感知-预测结合解决也是需要大量数据训练去得到合理的模型。
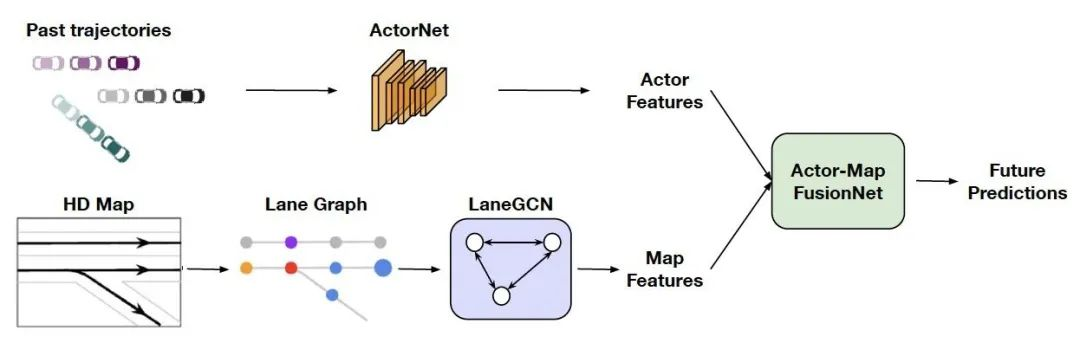
“Learning Lane Graph Representations for Motion Forecasting”
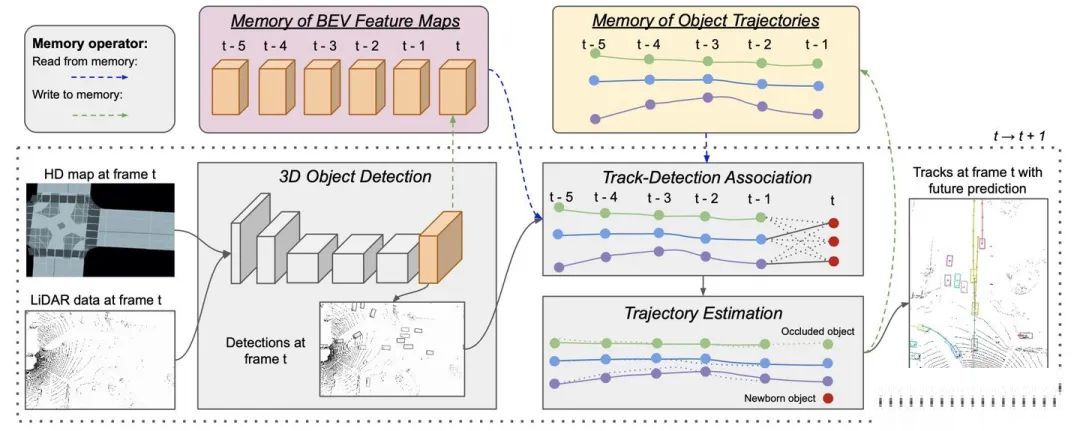
“PnPNet: End-to-End Perception and Prediction with
Tracking in the Loop”
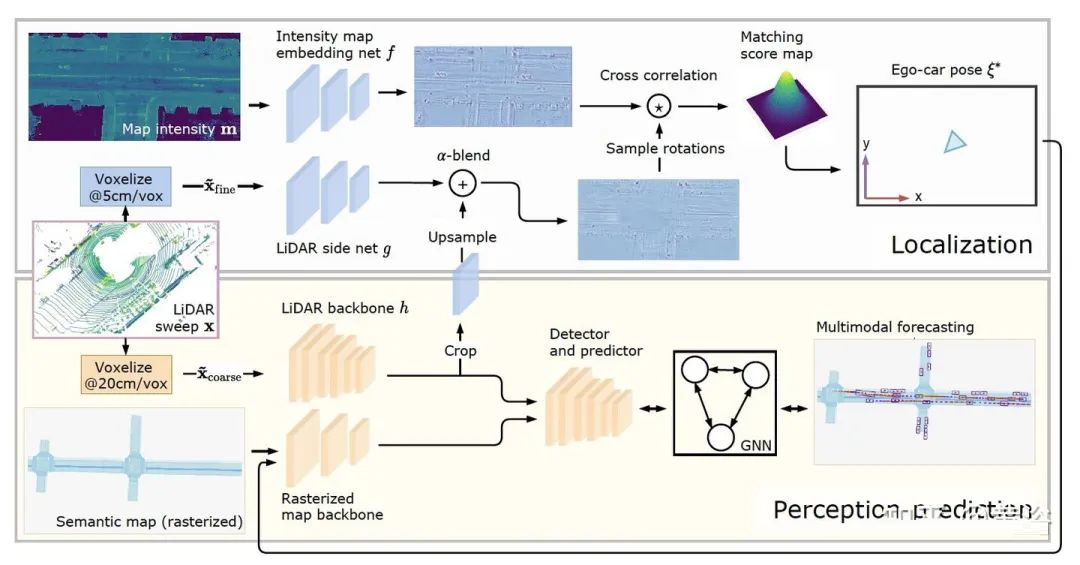
“Deep Multi-Task Learning for Joint Localization,
Perception, and Prediction”
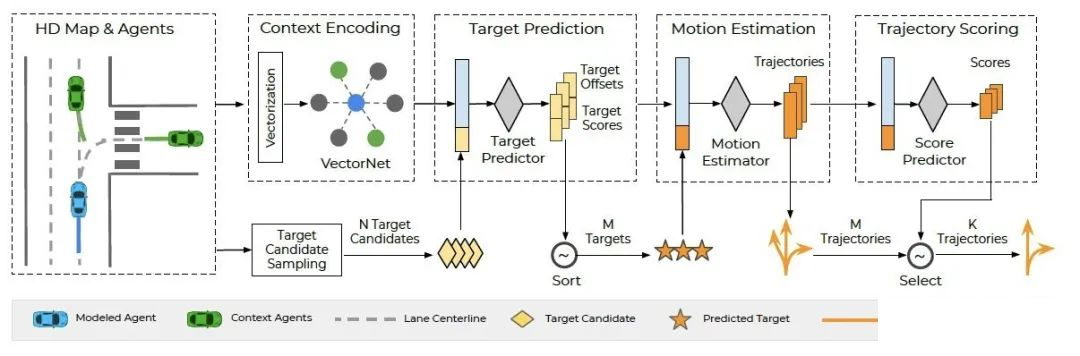
“TNT: Target-driven Trajectory Prediction”
4)规划:规划问题可以是传统的规则方法,也可以是强化学习或者模仿学习,其中强化学习也需要数据学习惩罚/奖励和策略。目前人们关心规划的地域化和个性化问题,这个比起感知的类似问题解决起来更加困难,比如激进还是保守地进行换道超车和并道汇入。最近有讨论合并预测和规划的解决方法,甚至包括地图定位一起建模,这个没有数据的“喂养”是很难成立的。
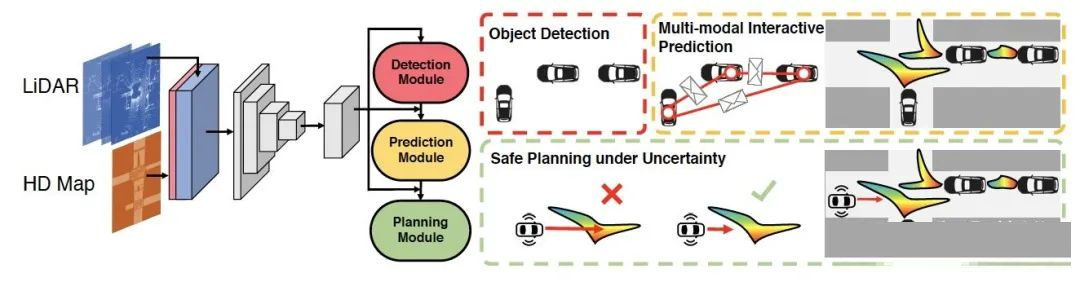
“DSDNet: Deep Structured self-Driving Network”
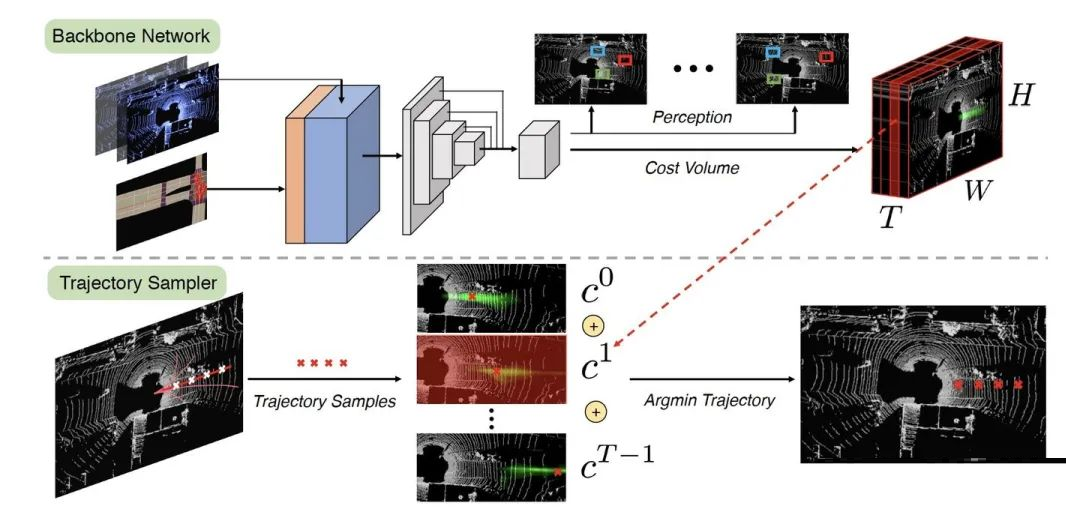
“End-to-end Interpretable Neural Motion Planner”
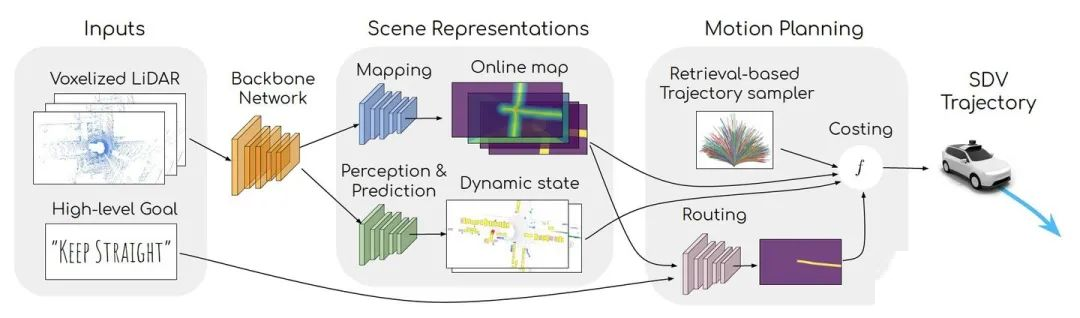
“MP3: A Unified Model to Map, Perceive,Predict and
Plan”
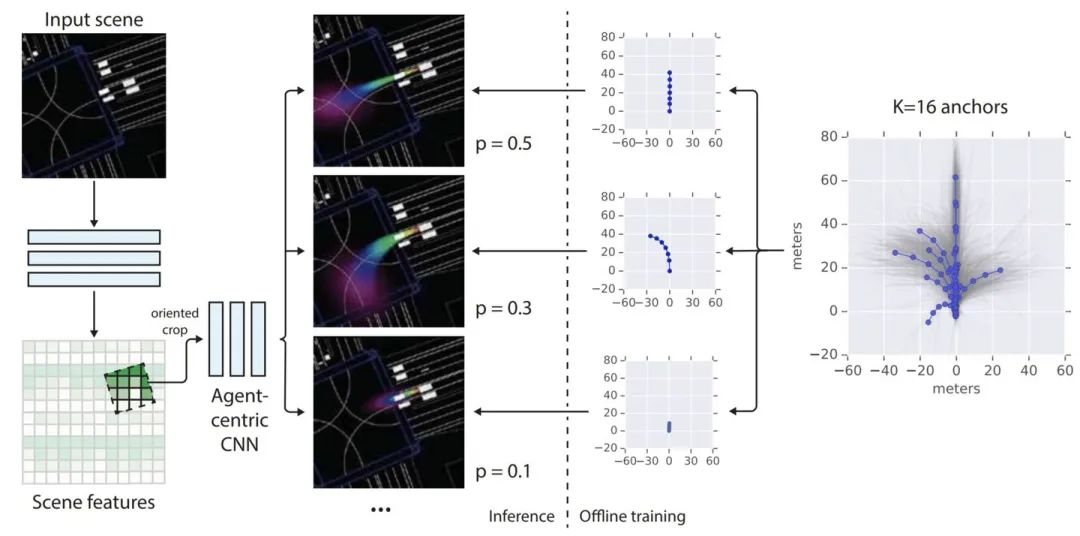
“Probabilistic Anchor Trajectory Hypotheses For Behavior
Prediction”
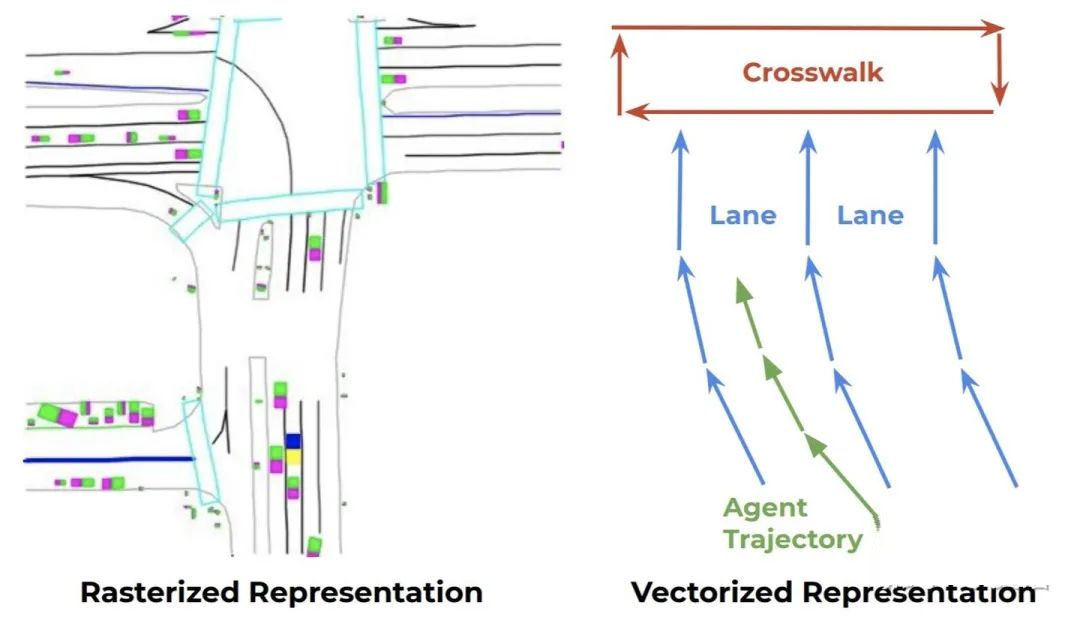
“VectorNet: Encoding HD Maps and Agent Dynamics From
Vectorized Representation”
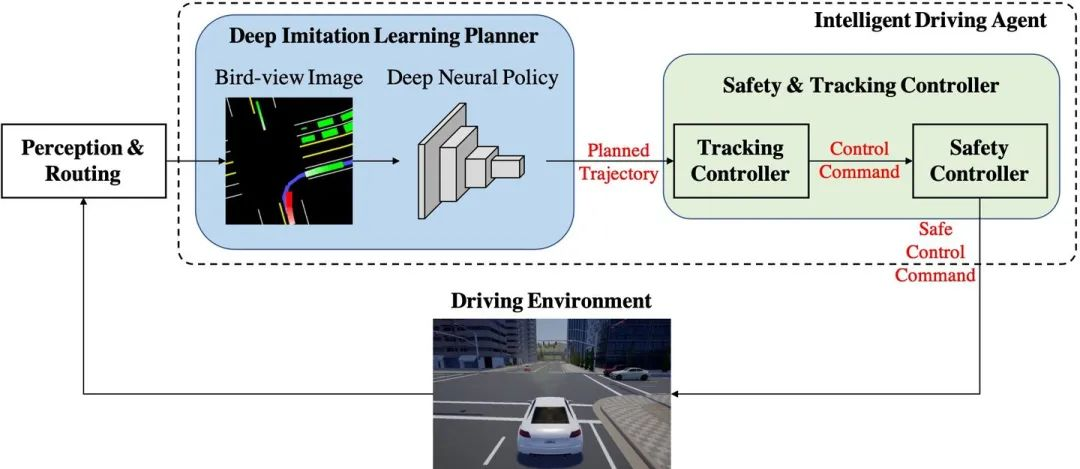
“Deep Imitation Learning for AV in Generic Urban Scenarios
with Enhanced Safety”
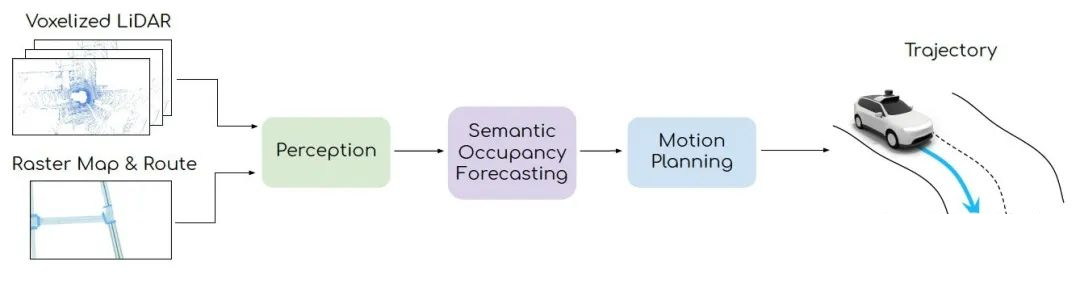
“Perceive, Predict, and Plan: Safe Motion Planning
Through Interpretable Semantic Rep”
5)控制:应该说,控制的传统方法相对成熟,不过并不是说数据驱动就没有价值,实际上规划和控制的确集成在一起建模也是大家感兴趣的领域,前面提到的强化学习和模仿学习同样是不错的解决手段。
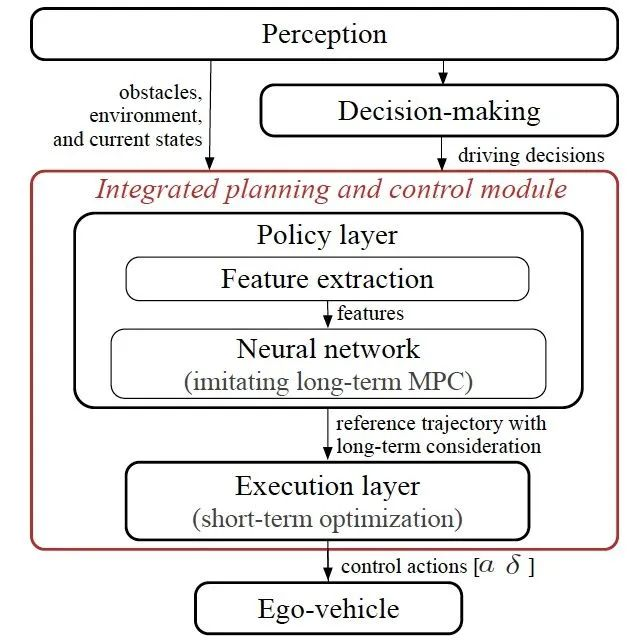
“A Fast Integrated Planning and Control Framework
for AV via Imitation Learning”
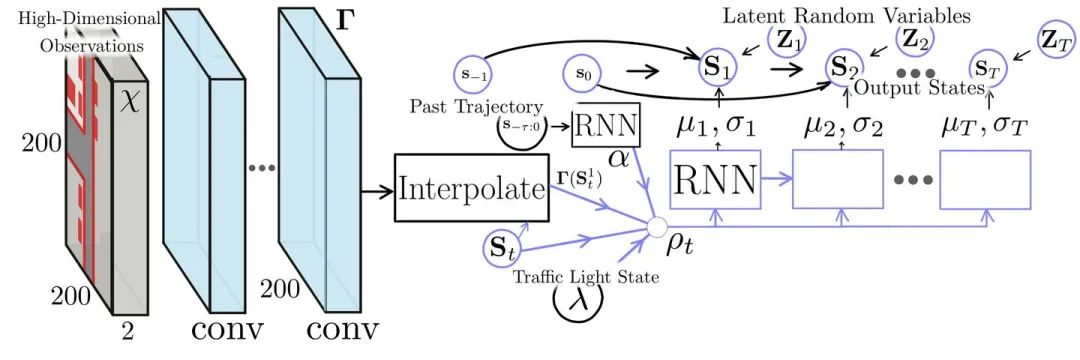
“Deep Imitative Models For Flexible Inference, Planning,
And Control”
6)传感器预处理:在进入感知模块之前,传感器的数据处理,比如污染检测、修补、去噪和增强等,传统的方法也会被数据驱动的机器学习方法取代。
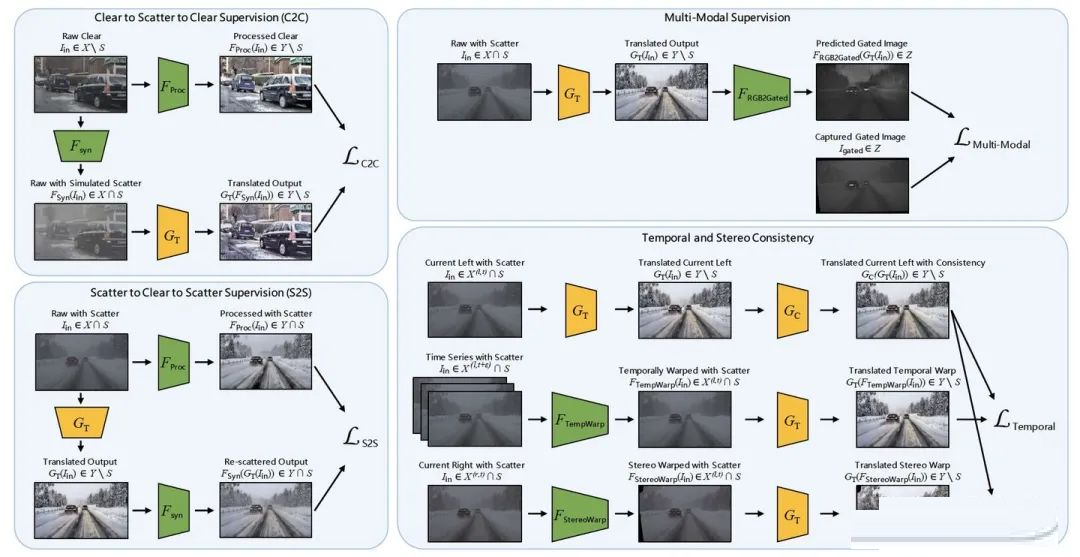
“ZeroScatter: Domain Transfer for Long Distance Imaging
and Visionthrough Scattering Media”
7)模拟仿真:模拟仿真需要对车辆/行人、传感器、交通环境和道路环境建模,有时候无法直接获得逼真的合成模型,特别是实际发生的交通事件,有时候不得不采用真实传感器数据来建模合成。下图是GAN-based的雨夜图像合成方法。
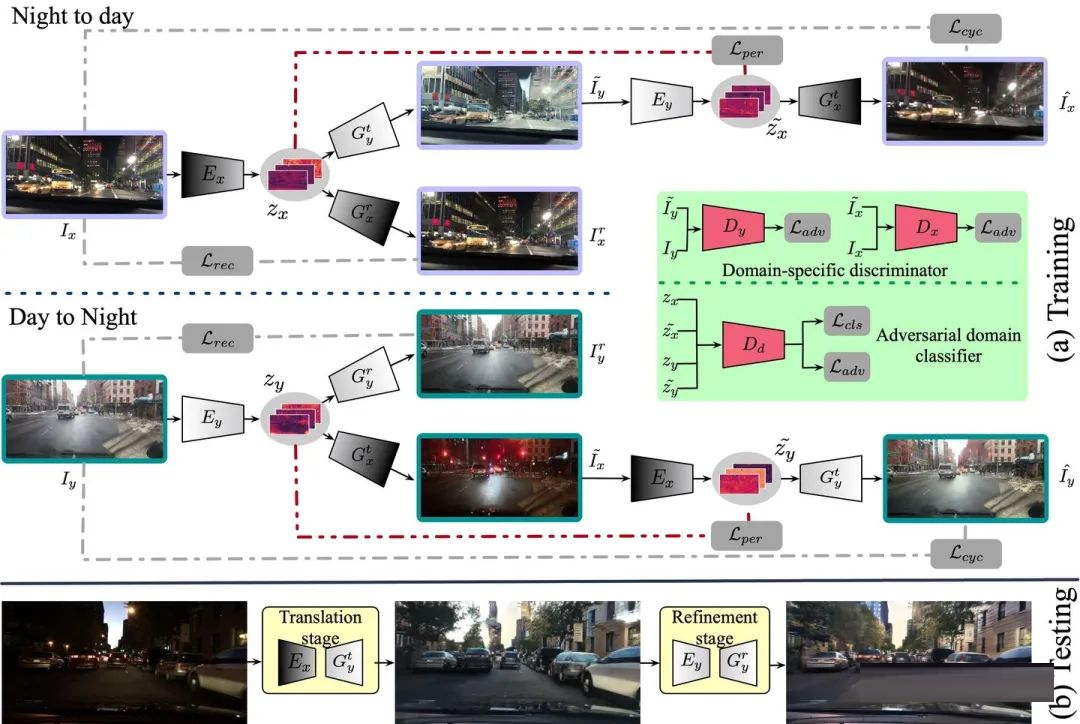
“ForkGAN: Seeing into the Rainy Night”
2 云计算平台的基建和大数据处理技术
数据闭环需要一个云计算/边缘计算平台和大数据的处理技术,这个不可能在单车或单机实现的。大数据云计算发展多年,在资源管理调度、数据批处理/流处理、工作流管理、分布式计算、系统状态监控和数据库存储等方面提供了数据闭环的基础设施支持,比如亚马逊AWS、微软Azure和谷歌云等。
Amazon Elastic Compute Cloud(EC2)是亚马逊云服务AWS的一部分,而Amazon
Elastic MapReduce(EMR) 是其大数据云平台,可使用多种开放源代码工具处理大量数据,例如数据流处理Apache
Spark、数据仓库Apache Hive和Apache HBase、数据流处理Apache Flink、数据湖Apache
Hudi和大数据分布式SQL查询引擎Presto。
下图是亚马逊云AWS提供的自动驾驶数据处理服务平台例子:其中标明1-10个任务环节
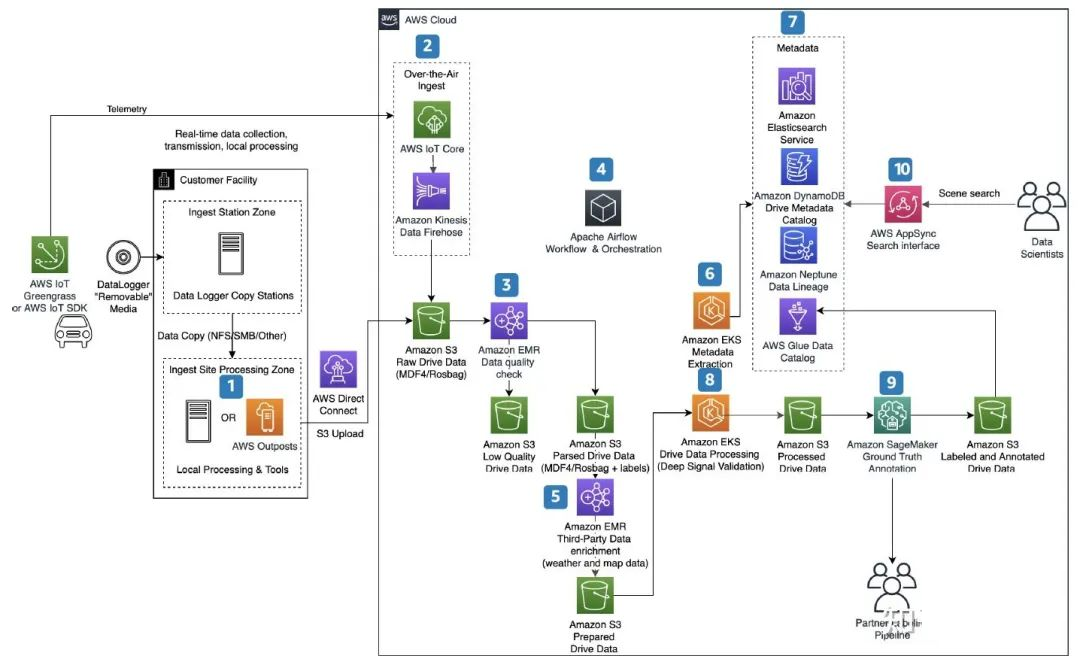
使用 AWS Outposts (运行本地 AWS 基础设施和服务)从车队中提取数据以进行本地数据处理。
使用 AWS IoT Core (将 IoT 设备连接到 AWS 云,而无需配置或管理服务器)和
Amazon Kinesis Data Firehose (将流数据加载到数据湖、数据存储和分析服务中)实时提取车辆T-box数据,该服务可以捕获和转换流数据并将其传输给
Amazon S3(AWS全球数据存储服务)、Amazon Redshift(用标准 SQL 在数据仓库、运营数据库和数据湖中查询和合并
EB 级结构化和半结构化数据)、Amazon Elasticsearch Service(部署、保护和运行
Elasticsearch,是一种在 Apache Lucene 上构建的开源 RESTful 分布式搜索和分析引擎)、通用
HTTP 终端节点和服务提供商(如 Datadog、New Relic、MongoDB 和 Splunk),这里Amazon
Kinesis 提供的功能Data Analytics, 可通过 SQL 或 Apache Flink
(开源的统一流处理和批处理框架,其核心是分布流处理数据引擎)的实时处理数据流。
删除和转换低质量数据。
使用 Apache Airflow (开源工作流管理工具)安排提取、转换和加载 (ETL) 作业。
基于 GPS 位置和时间戳,附加天气条件来丰富数据。
使用 ASAM OpenSCENARIO (一种驾驶和交通模拟器的动态内容文件格式)提取元数据,并存储在Amazon
DynamoDB (NoSQL 数据库服务)和 Amazon Elasticsearch Service中。
在 Amazon Neptune (图形数据库服务,用于构建查询以有效地导航高度互连数据集)存储数据序列,并且使用
AWS Glue Data Catalog(管理ETL服务的AWS Glue提供数据目录功能)对数据建立目录。
处理驾驶数据并深度验证信号。
使用 Amazon SageMaker Ground Truth (构建训练数据集的标记工具用于机器学习,包括
3D 点云、视频、图像和文本)执行自动数据标记,而Amazon SageMaker 整合ML功能集,提供基于
Web 的统一可视化界面,帮助数据科学家和开发人员快速准备、构建、训练和部署高质量的机器学习 (ML)
模型。
AWS AppSync 通过处理与 AWS DynamoDB、AWS Lambda(事件驱动、自动管理代码运行资源的计算服务平台)
等数据源之间连接任务来简化数据查询/操作GraphQL API 的开发,在此使用是为特定场景提供搜索功能。
下图是AWS给出的一个自动驾驶数据流水线框架:数据收集、注入和存储、模型训练和部署;其中Snowball是AWS的边缘计算系列之一,负责车辆和AWS
S3之间的数据传输;其他还有两个,是AWS Snowcone和 AWS Snowmobile。
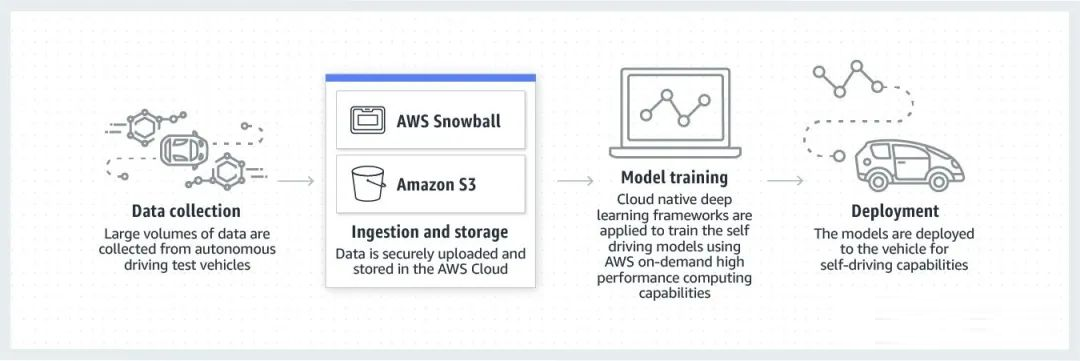
可以看到,AWS使用了数据存储S3、数据传输Snowball、数据库DynamoDB、数据流处理Flink和Spark、搜索引擎Elasticsearch、工作流管理Apache
Airflow和机器学习开发平台SageMaker等。
其他开源的使用,比如流处理的实时数据馈送平台Apache Kafka、资源管理&调度Apache
Mesos和分布NoSQL数据库Apache Cassandra。
如图是国内自动驾驶公司Momenta基于亚马逊AWS建立的系统架构实例图:
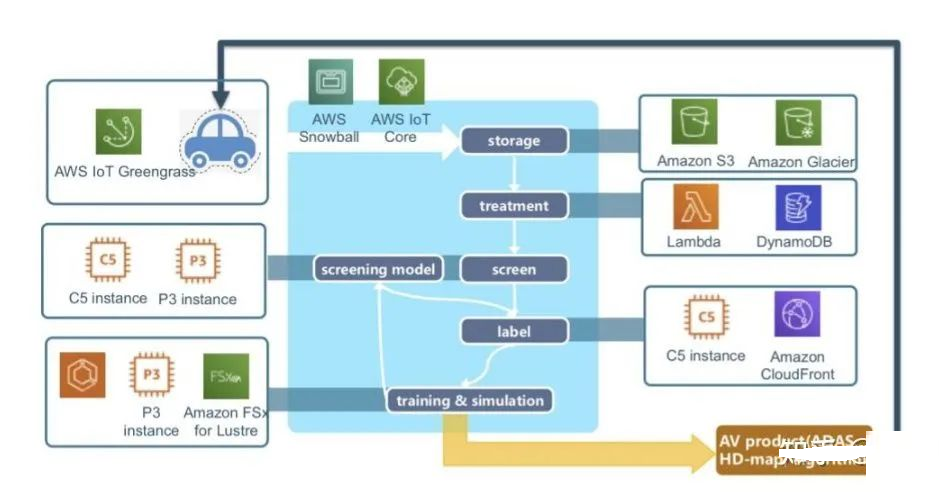
其中AWS IoT Greengrass 提供边缘计算及机器学习推理功能,可以实时处理车辆中的本地规则和事件,同时最大限度地降低向云传输数据的成本。
其中P3实例和C5实例是Amazon EC2提供的。Amazon CloudFront是AWS的CDN,Amazon
Glacier是在线文件存储服务,而Amazon FSx for Lustre 是可扩展的高性能文件存储系统。
除此之外,亚马逊指出的,Momenta采用的AWS服务还包括:监控可观测性服务Amazon CloudWatch、关系数据库Amazon
Relational Database Service (Amazon RDS)、实时流数据处理和分析服务Amazon
Kinesis(包括Video Streams、Data Streams、Data Firehose和Data
Analytics)和消息队列服务Amazon Simple Queue Service (Amazon
SQS)等。
最近Momenta还采用Amazon Elastic Kubernetes Service (EKS)
运行容器Kubernetes。此外亚马逊也推荐了Kubernetes服务,AWS Fargate。
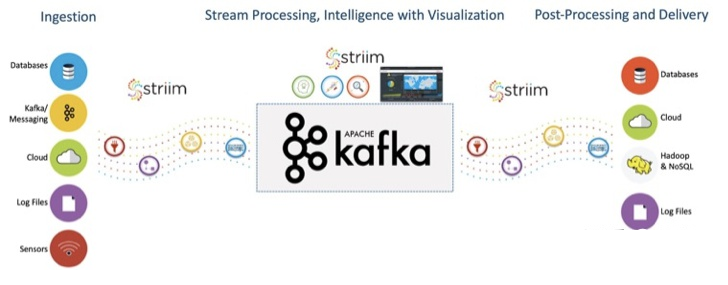
Apache Kafka
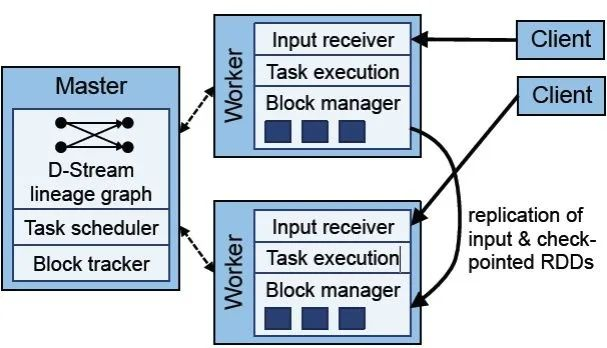
Apache Spark
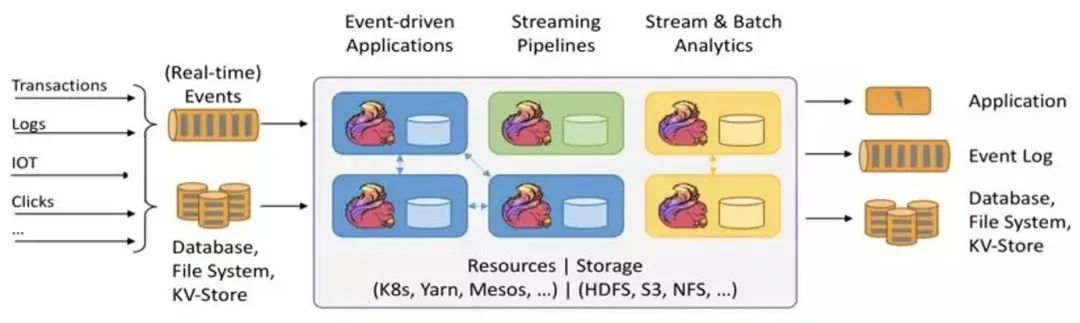
Apache Flink
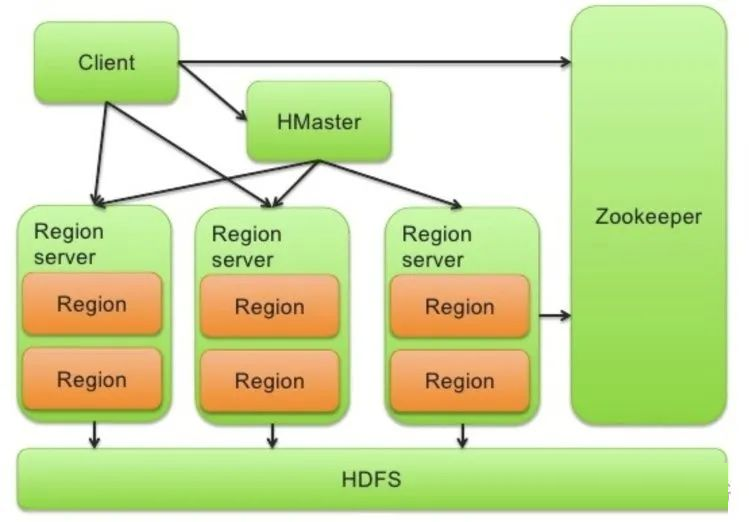
Apache HBase
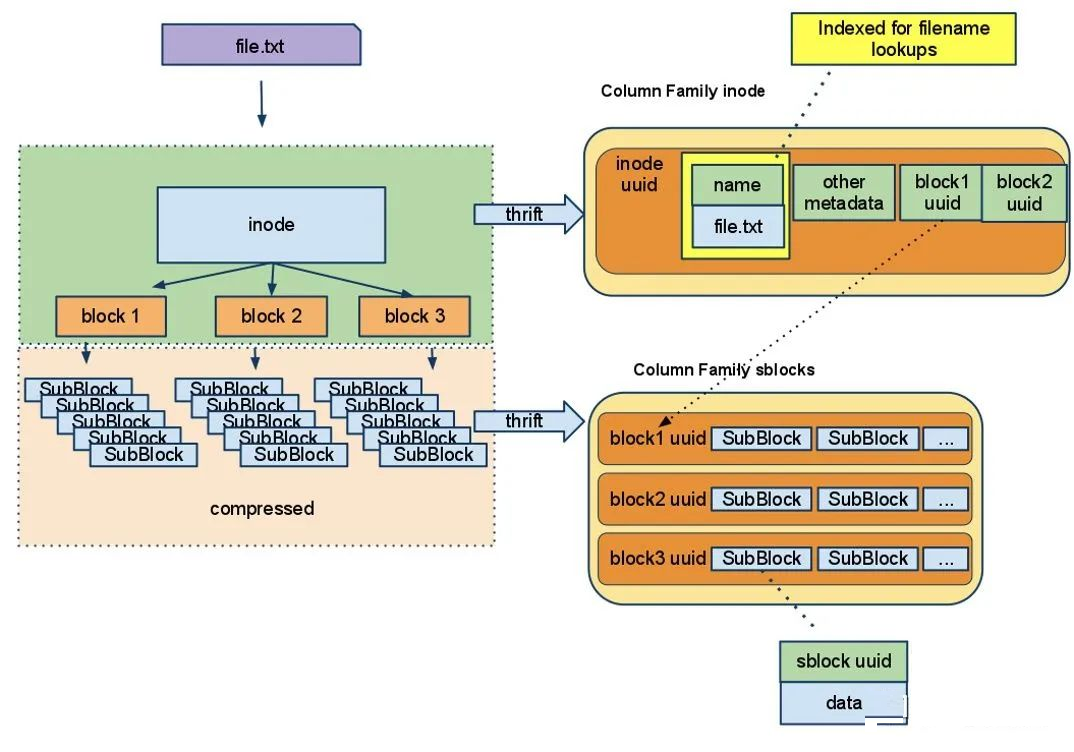
Apache Cassandra
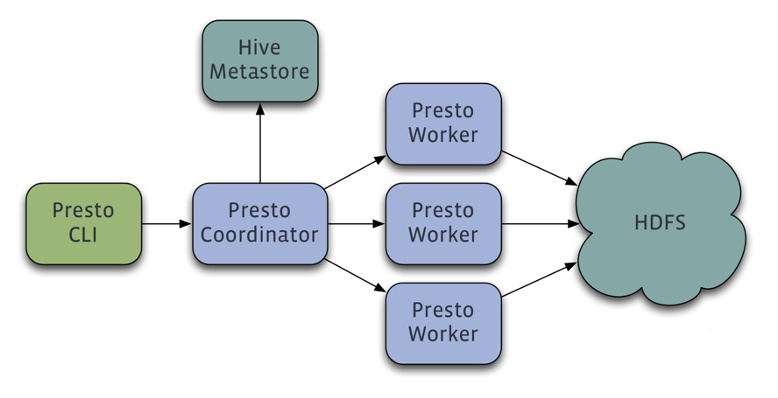
Presto
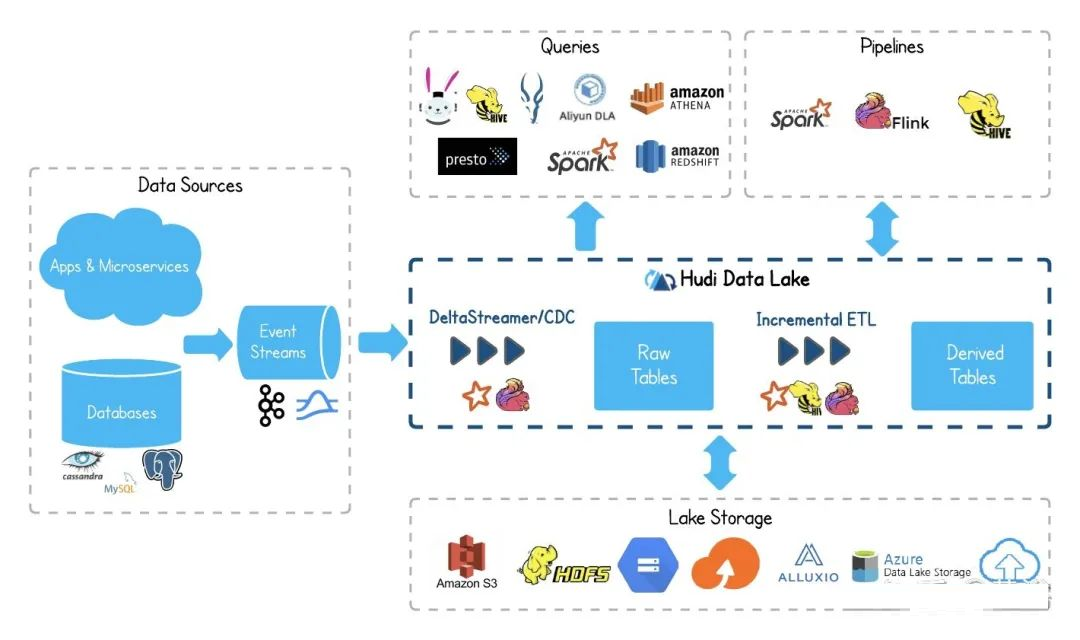
Apache Hudi
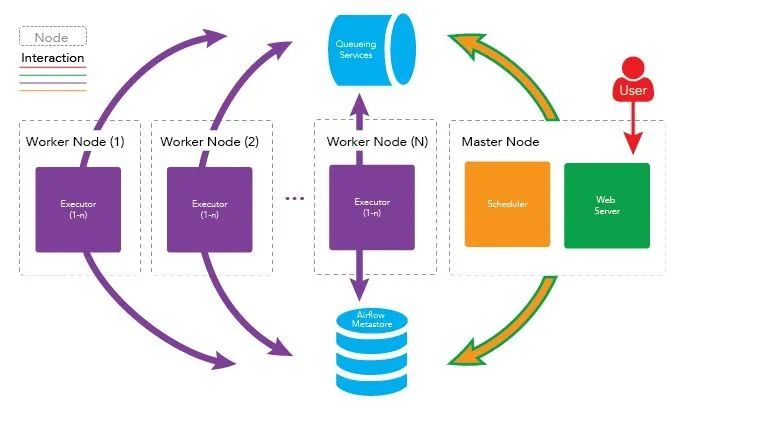
Apache Airflow
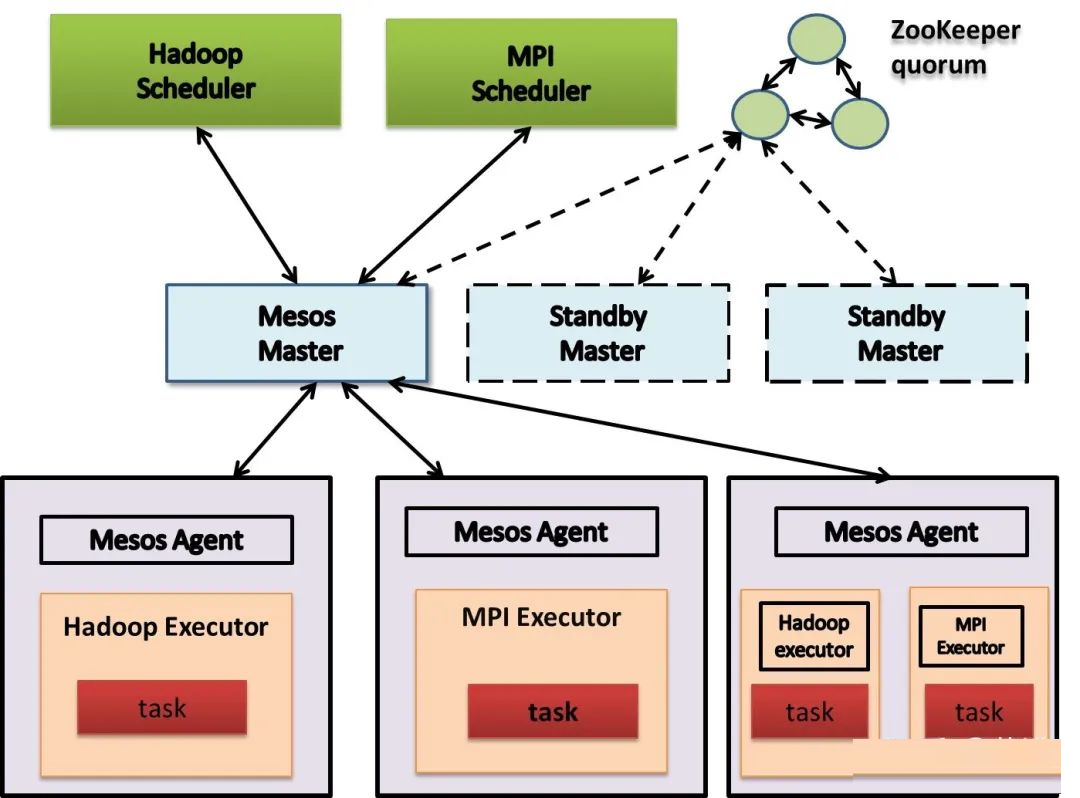
Apache Mesos
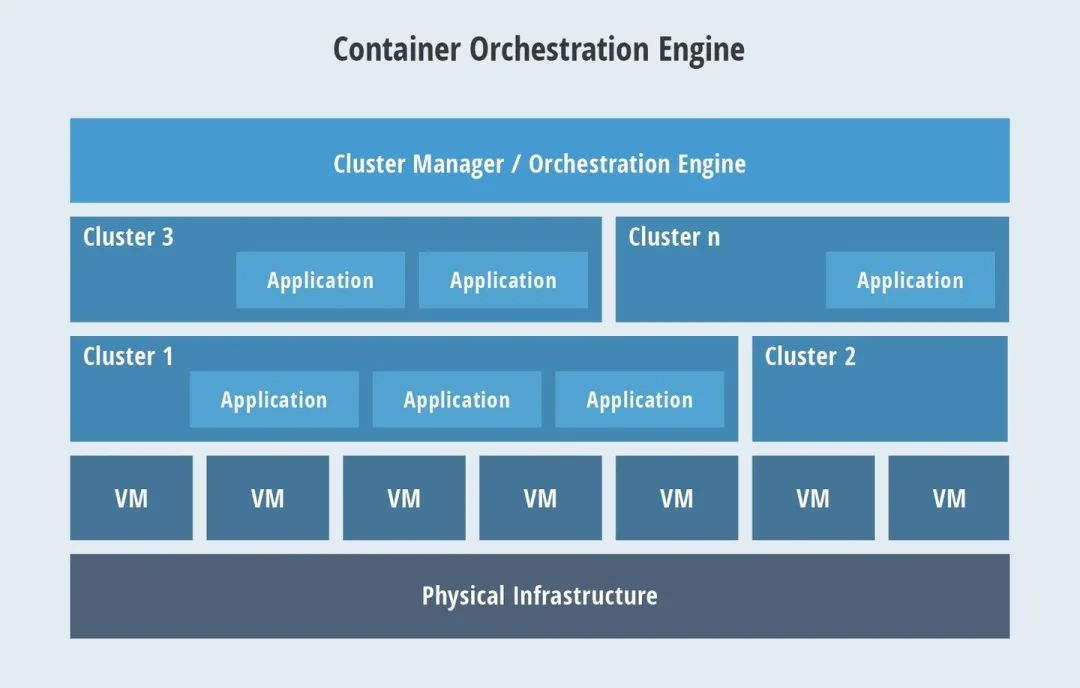
Kubernetes
3 训练数据标注工具
其实AWS的机器学习平台本身也提供了数据标注工具Amazon SageMaker Ground Truth。
如图是微软开源标注工具VOTT(Video Object Tagging Tool):
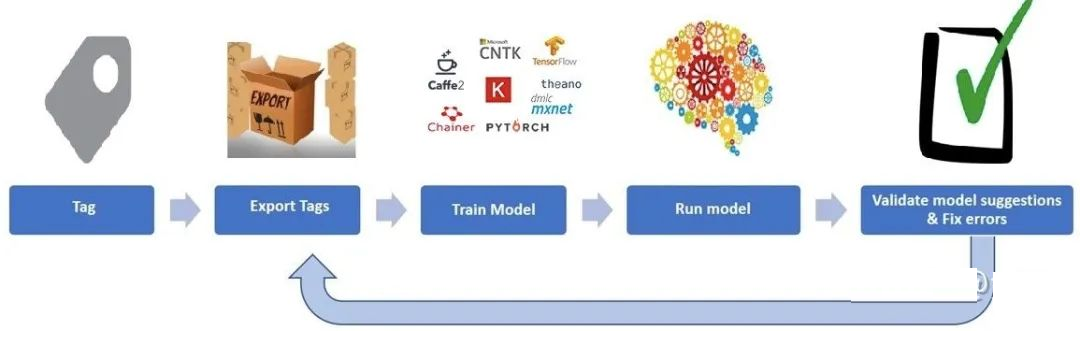
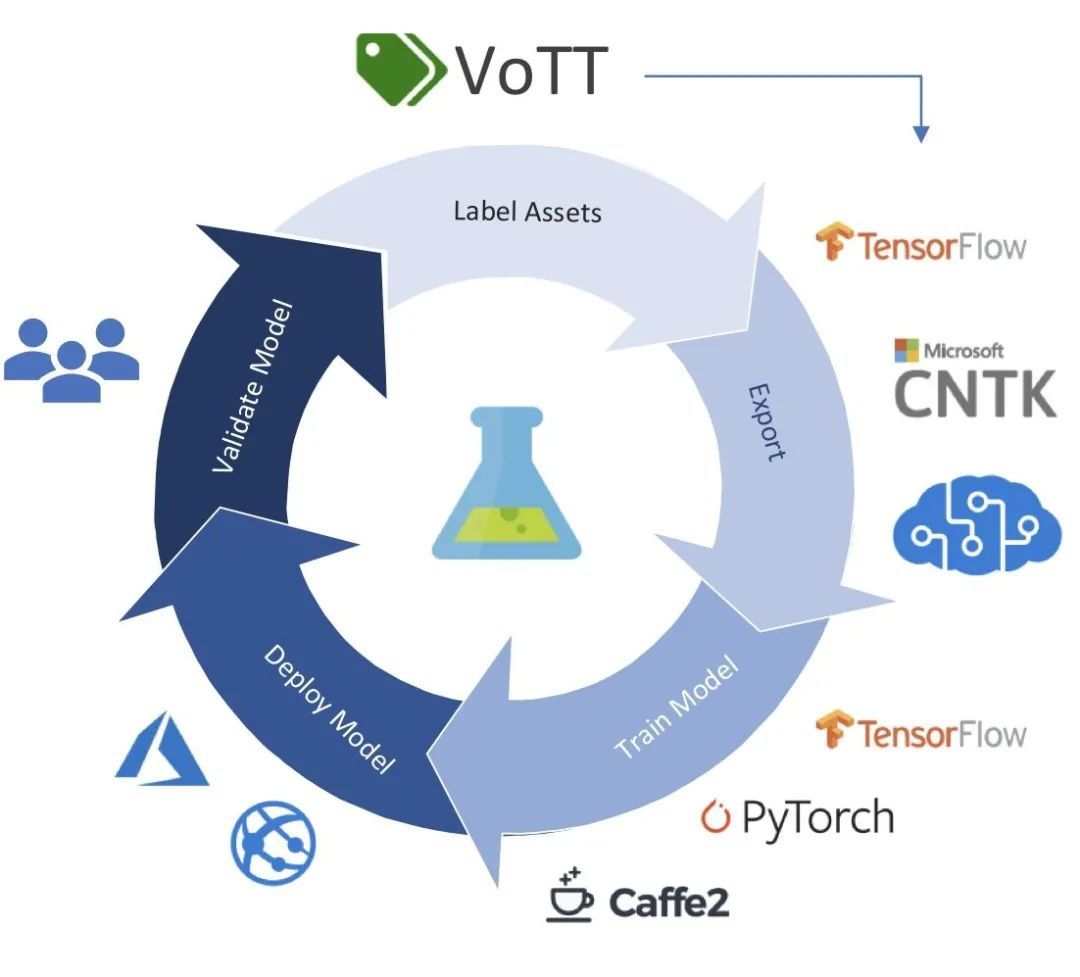
标注工具可以是全自动、半自动和手工等3类。
比如人工标注工具:摄像头图像LabelMe和激光雷达点云PCAT
还有半自动标注工具:摄像头CVAT、VATIC,激光雷达3D BAT、SAnE,图像点云融合Latte
自动标注工具:基本没有开源(商用也没有吧)的工具可用。
这里有一些自动标注方面的论文:
“Beat the MTurkers: Automatic Image Labeling from
Weak 3D Supervision“
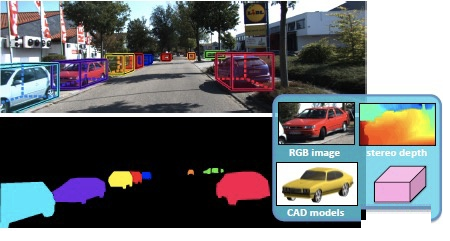
“Auto-Annotation of 3D Objects via ImageNet“
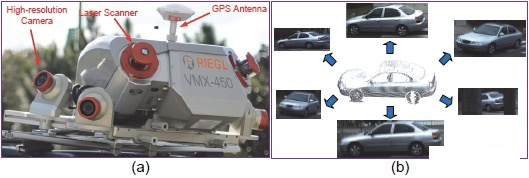
“Offboard 3D Object Detection from Point Cloud Sequences“
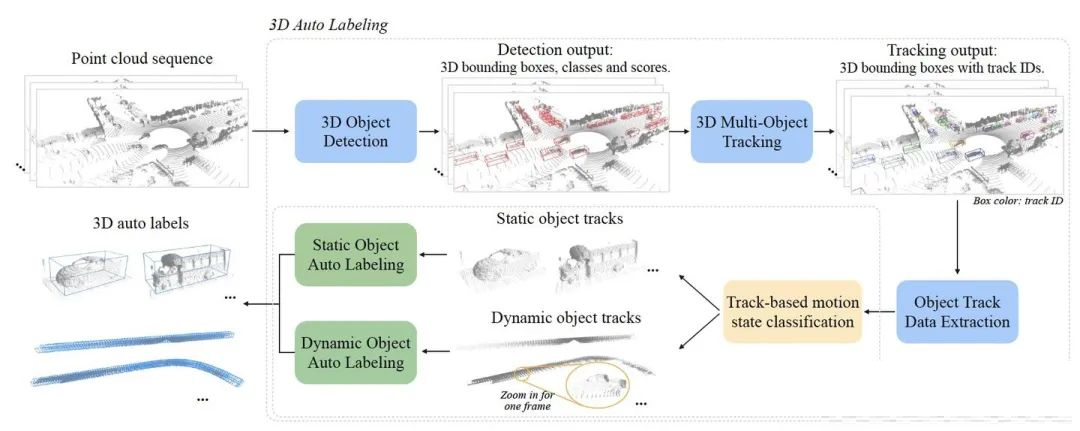
这里是Nvidia在会议报告中给出的端到端标注流水线:它需要人工介入
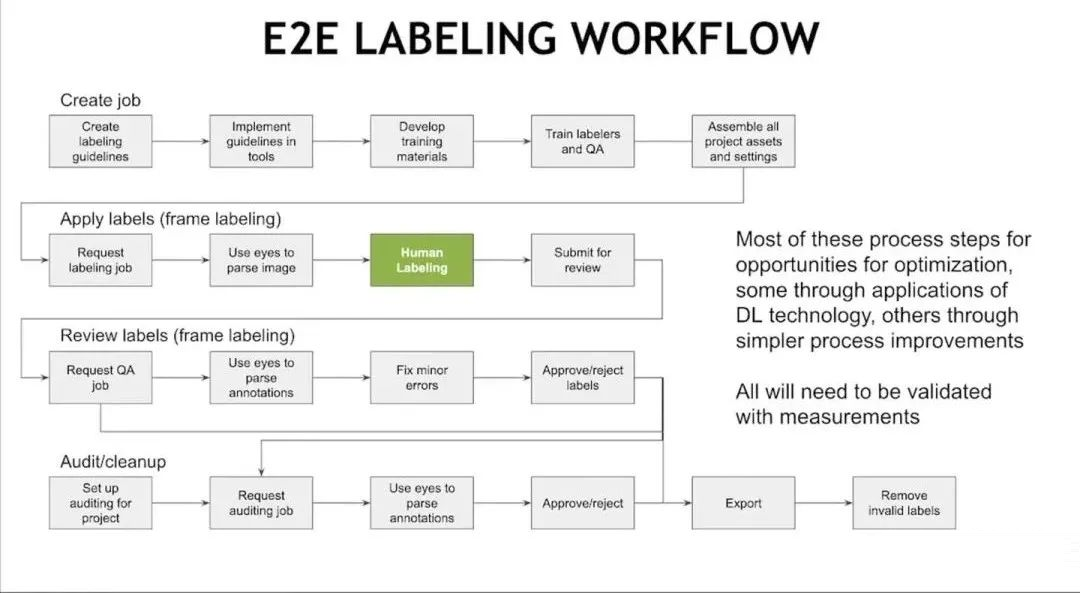
在这里顺便提一下“数据可视化”的问题,各种传感器数据除了标注,还需要一个重放、观察和调试的平台。如图是Uber提供的开源可视化工具
Autonomous Visualization System (AVS):
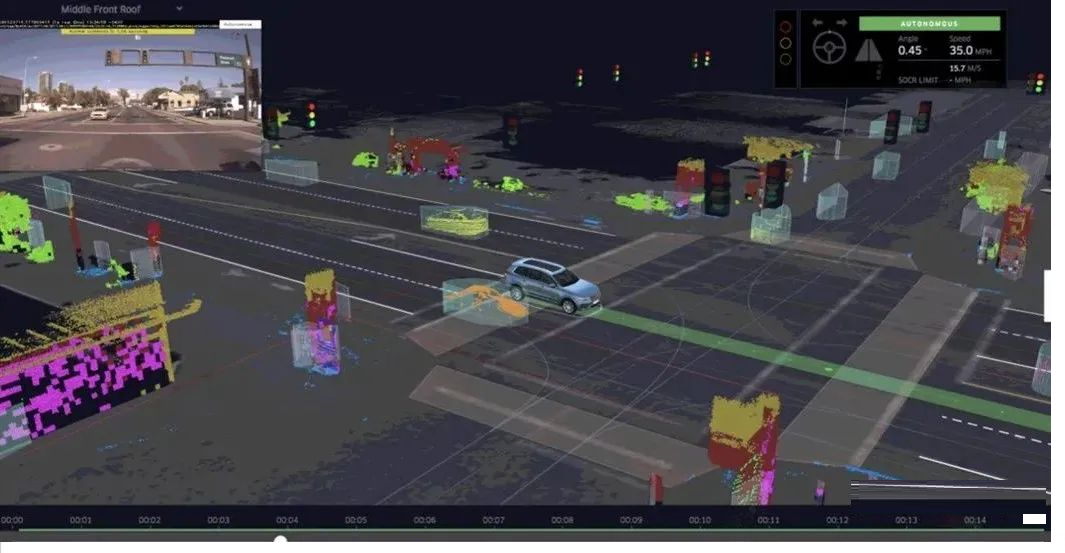
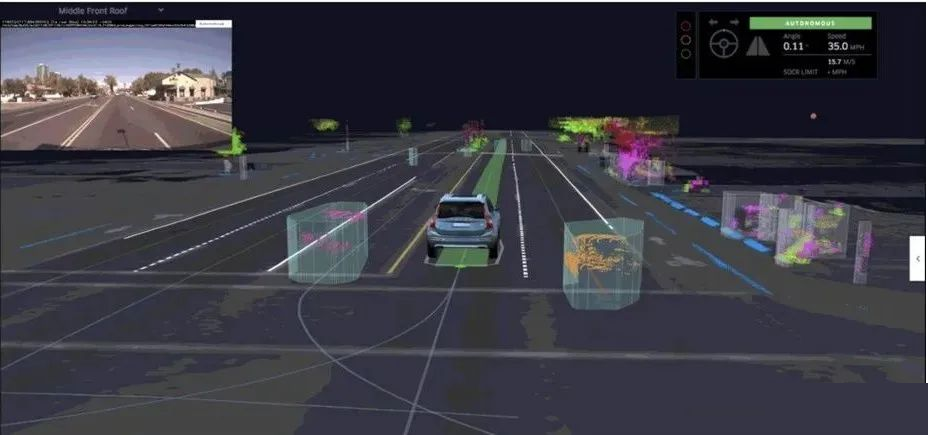
其中”XVIZ“是提出的自动驾驶数据实时传输和可视化协议:
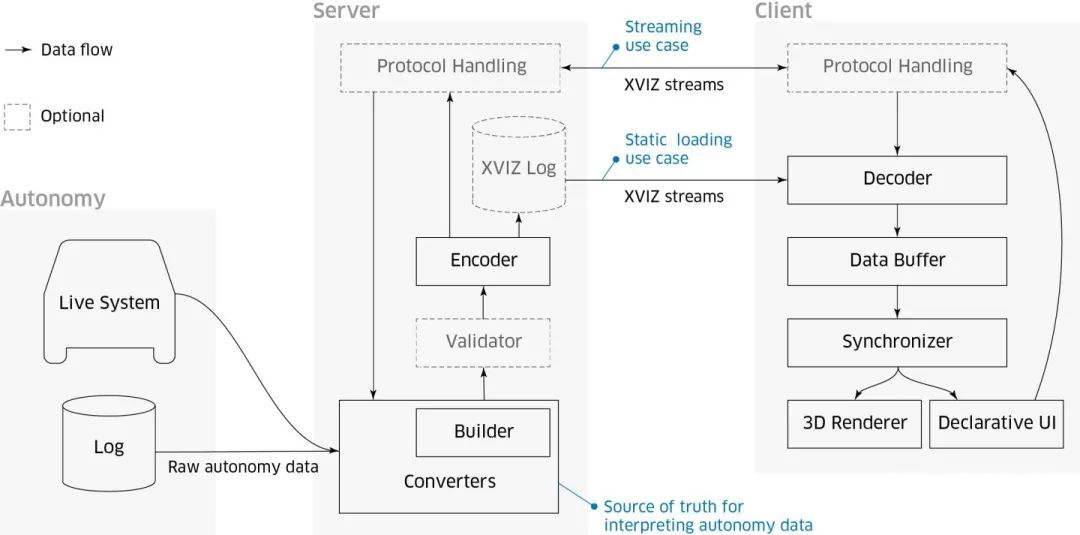
另外,“streetscape.gl“是一个可视化工具包,在XVIZ 协议编码自动驾驶和机器人数据。它提供了一组可组合的
React 组件,对 XVIZ 数据进行可视化和交互。
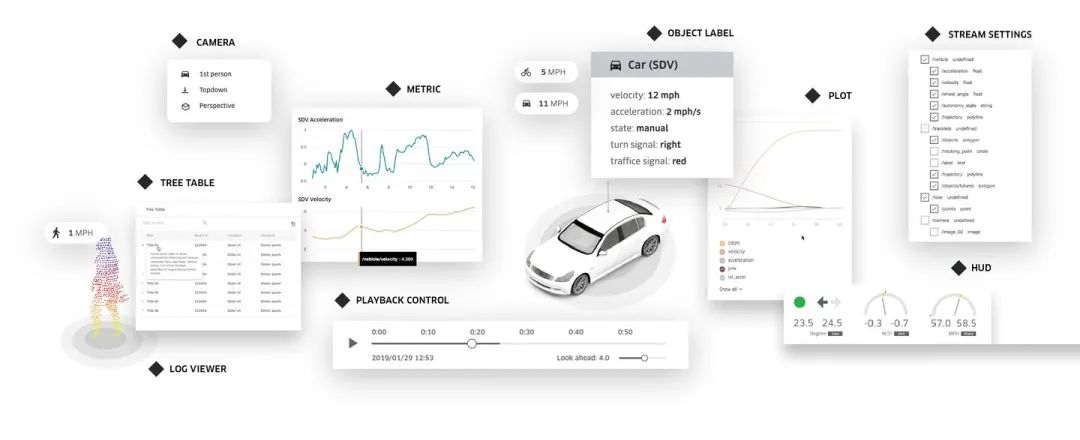
4 大型模型训练平台
模型训练平台,主要是机器学习(深度学习)而言,前面亚马逊AWS提供了自己的ML平台SageMaker。我们知道最早有开源的软件Caffe,目前最流行的是Tensorflow和Pytorch(Caffe2并入)。
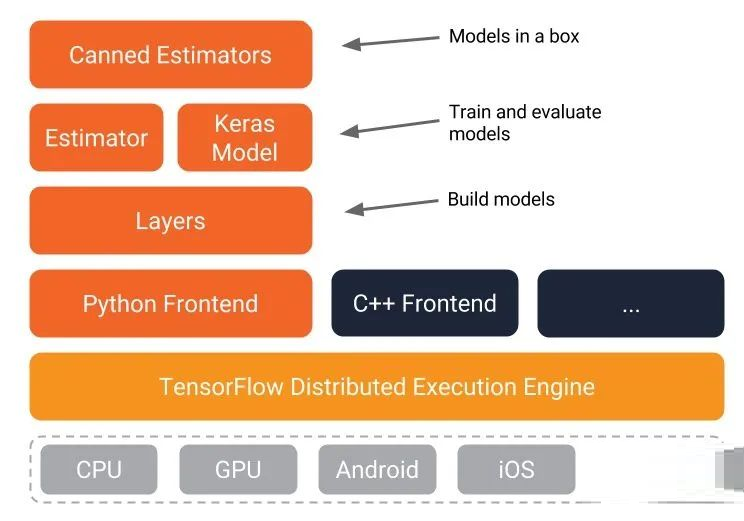
Tensorflow
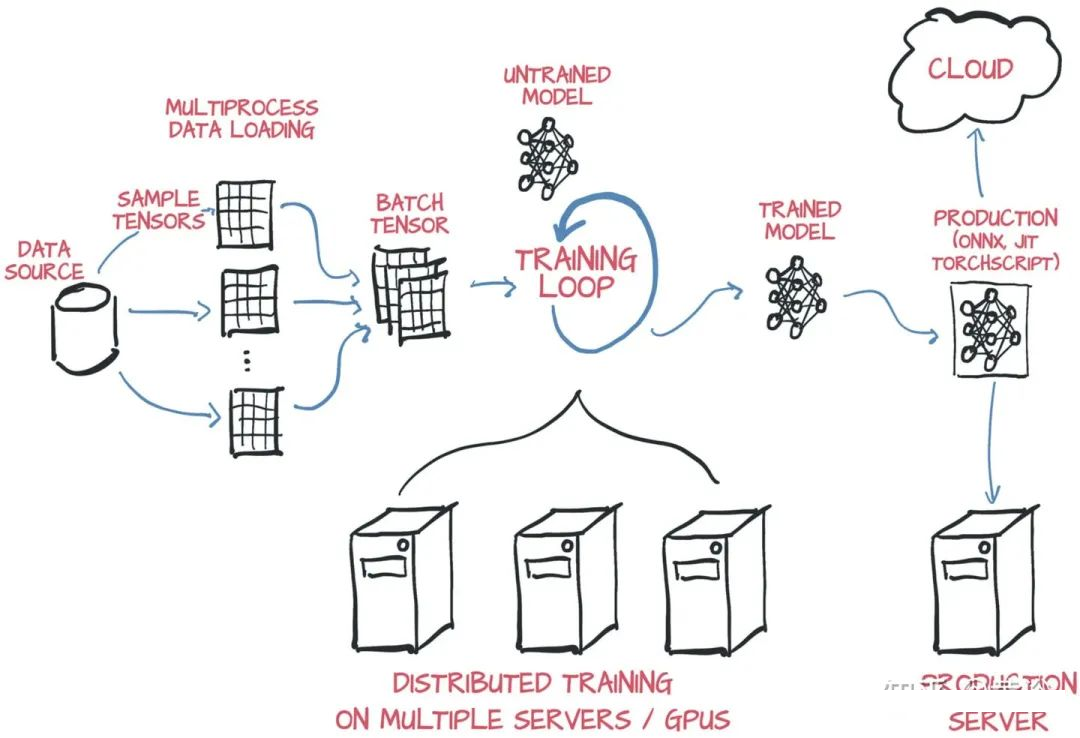
Pytorch
在云平台部署深度学习模型训练,一般采用分布式。按照并行方式,分布式训练一般分为数据并行和模型并行两种。当然,也可采用数据并行和模型并行的混合。
模型并行:不同GPU负责网络模型的不同部分。例如,不同网络层被分配到不同的GPU,或者同一层不同参数被分配到不同GPU。
数据并行:不同GPU有模型的多个副本,每个GPU分配不同的数据,将所有GPU计算结果按照某种方式合并。
模型并行不常用,而数据并行涉及各个GPU之间如何同步模型参数,分为同步更新和异步更新。同步更新等所有GPU的梯度计算完成,再计算新权值,同步新值后,再进行下一轮计算。异步更新是每个GPU梯度计算完无需等待,立即更新权值,然后同步新值进行下一轮计算。
分布式训练系统包括两种架构:Parameter Server Architecture(PS,参数服务器)和Ring
-AllReduce Architecture(环-全归约)。
如下图是PS结构图:
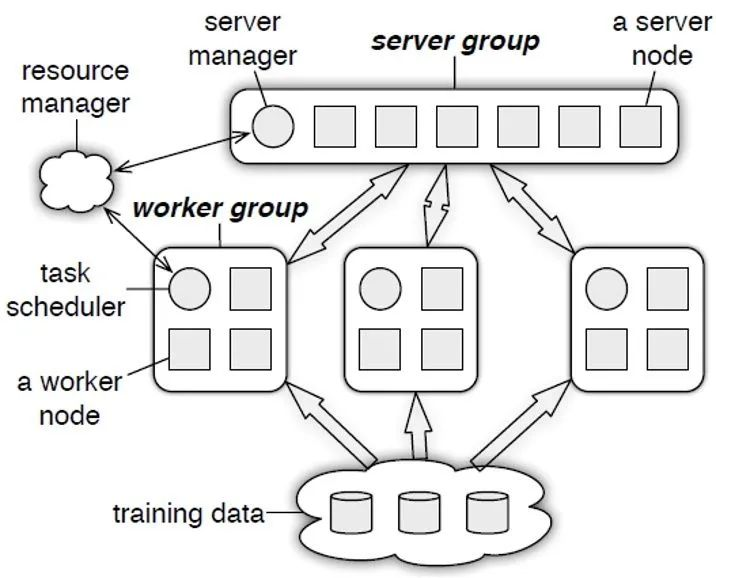
这个图是Ring AllReduce的架构图:
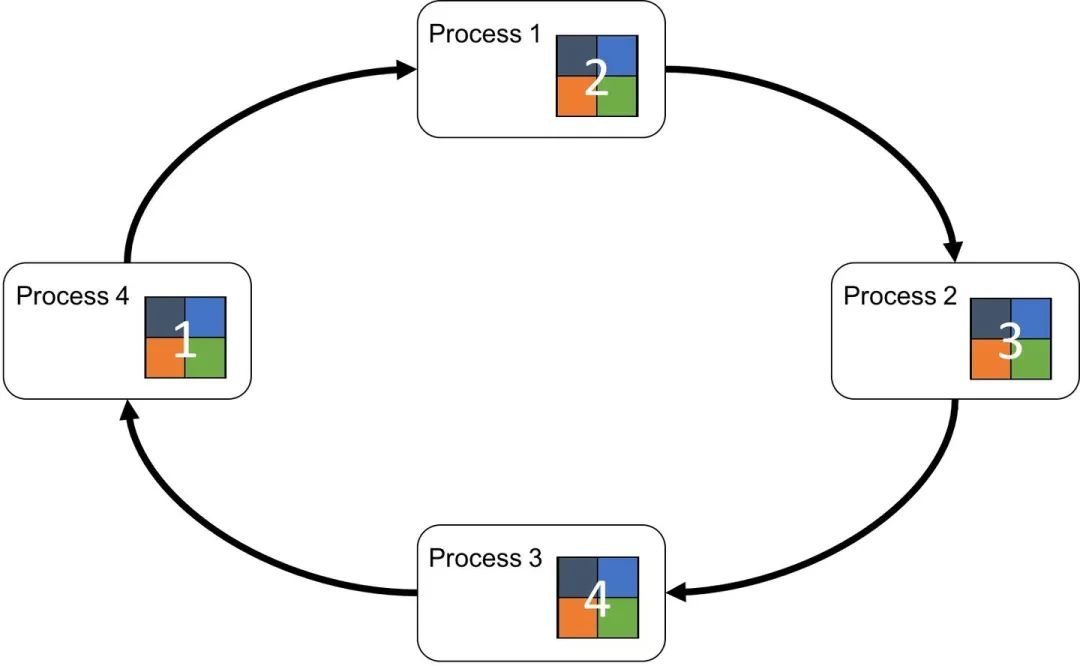
Pytorch现在和多个云平台建立合作关系,可以安装使用。比如AWS,在AWS Deep Learning
AMIs、AWS Deep Learning Containers和Amazon SageMaker,都可以训练Pytorch模型,最后采用TorchServe进行部署。
Pytorch提供两种方法在多GPU平台切分模型和数据:
DataParallel
distributedataparallel
DataParallel更易于使用。不过,通信是瓶颈,GPU利用率通常很低,而且不支持分布式。DistributedDataParallel支持模型并行和多进程,单机/多机都可以,是分布训练。
PyTorch 自身提供几种加速分布数据并行的训练优化技术,如 bucketing gradients、overlapping
computation with communication 以及 skipping gradient
synchronization 等。
Tensorflow在模型设计和训练使用也方便,可以使用高阶 Keras API;对于大型机器学习训练任务,使用
Distribution Strategy API 在不同的硬件配置上进行分布式训练,而无需更改模型定义。
其中Estimator API 用于编写分布式训练代码,允许自定义模型结构、损失函数、优化方法以及如何进行训练、评估和导出等内容,同时屏蔽与底层硬件设备、分布式网络数据传输等相关的细节。
tf.distribute.MirroredStrategy支持在一台机器的多个 GPU 上进行同步分布式训练。该策略会为每个
GPU 设备创建一个副本。模型中的每个变量都会在所有副本之间进行镜像。这些变量将共同形成一个名为MirroredVariable的单个概念变量。这些变量会通过应用相同的更新彼此保持同步。
tf.distribute.experimental.MultiWorkerMirroredStrategy与MirroredStrategy非常相似。它实现了跨多个工作进程的同步分布式训练,而每个工作进程可能有多个
GPU。与MirroredStrategy类似,它也会跨所有工作进程在每个设备的模型中创建所有变量的副本。
tf.distribute.experimental.ParameterServerStrategy支持在多台机器上进行参数服务器PS训练。在此设置中,有些机器会被指定为工作进程,有些会被指定为参数服务器。模型的每个变量都会被放在参数服务器上。计算会被复制到所有工作进程的所有
GPU 中。(注:该策略仅适用于 Estimator API。)
5 模型测试和检验
模型的测试和检验可以分成多种方式:
一是仿真测试检验。建立仿真测试环境,比如开源的一些软件平台:
Carla
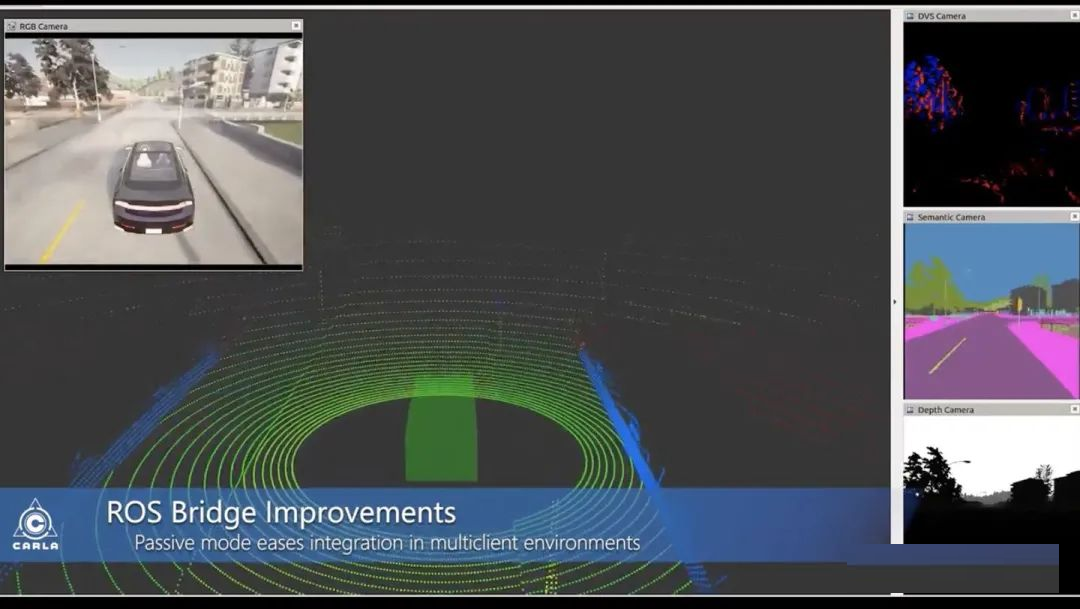
AirSim
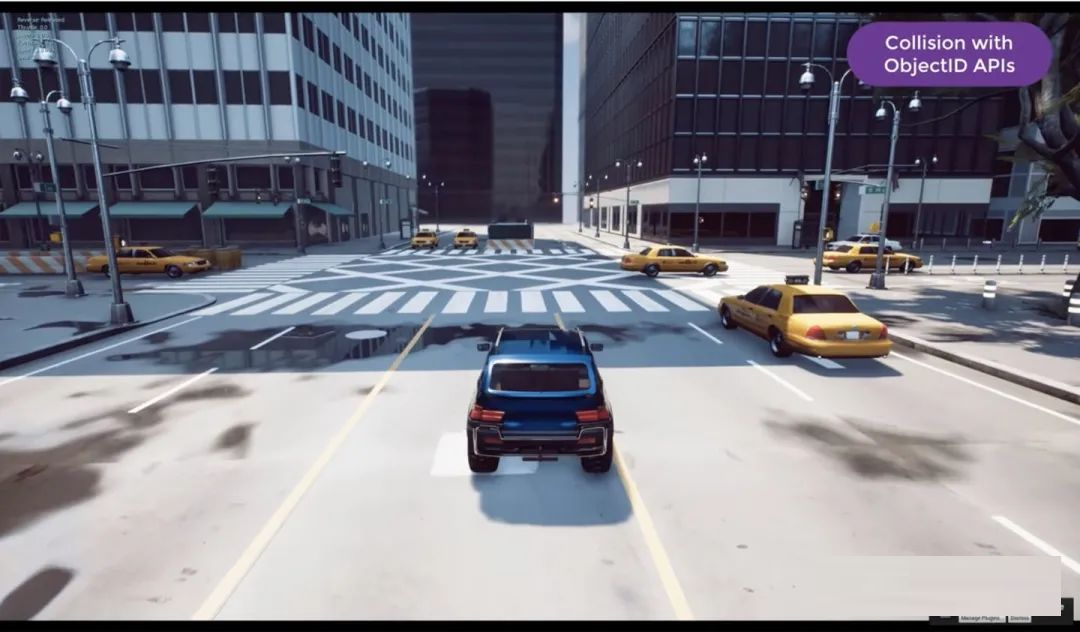
LGSVL
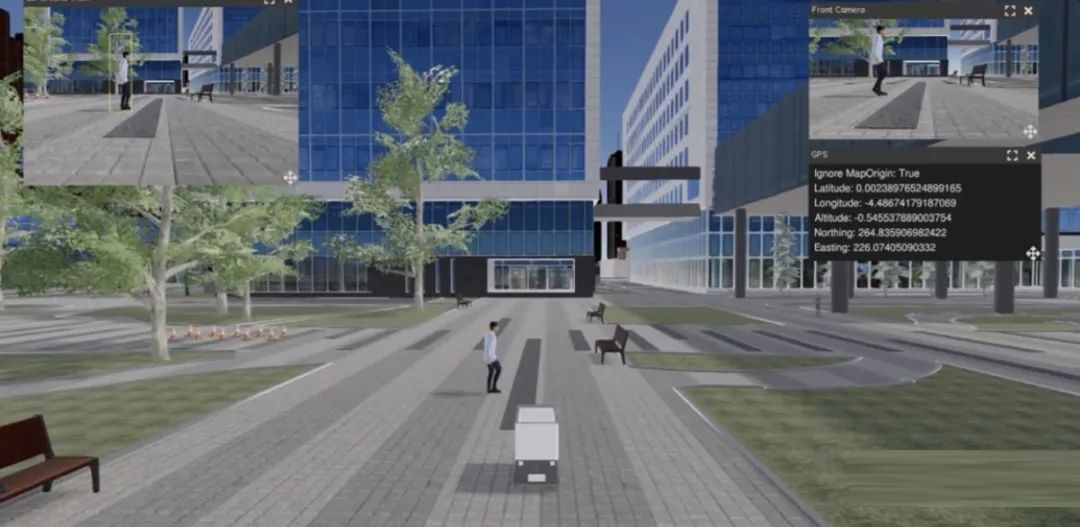
还有一些成熟的商用软件,也可以构建仿真测试环境:Prescan和VTD。存在一些仿真子模块,比如开源的交通流仿真方面SUMO,商用的动力学仿真方面CarSim、Trucksim和Carmaker等。测试方式包括模型在环(MIL)、软件在环(SIL)、硬件在环(HIL)和整车在环(VIL)等。传感器的仿真,特别是摄像头的图像生成,除了图形学的渲染方式,还有基于机器学习的方式。
这里列出Uber ATG发表的一系列仿真建模论文:
“LiDARsim: Realistic LiDAR Simulation by Leveraging
the Real World“
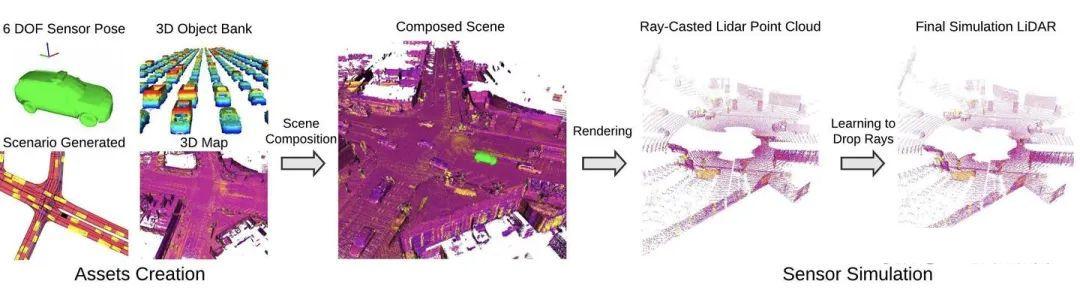
”S3: Neural Shape, Skeleton, and Skinning Fields for
3D Human Modeling“
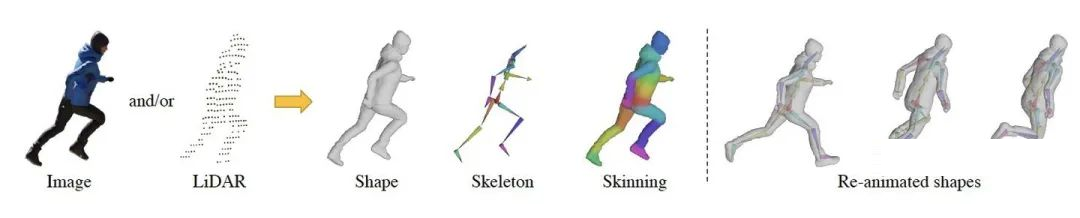
”SceneGen: Learning to Generate Realistic Traffic
Scenes“
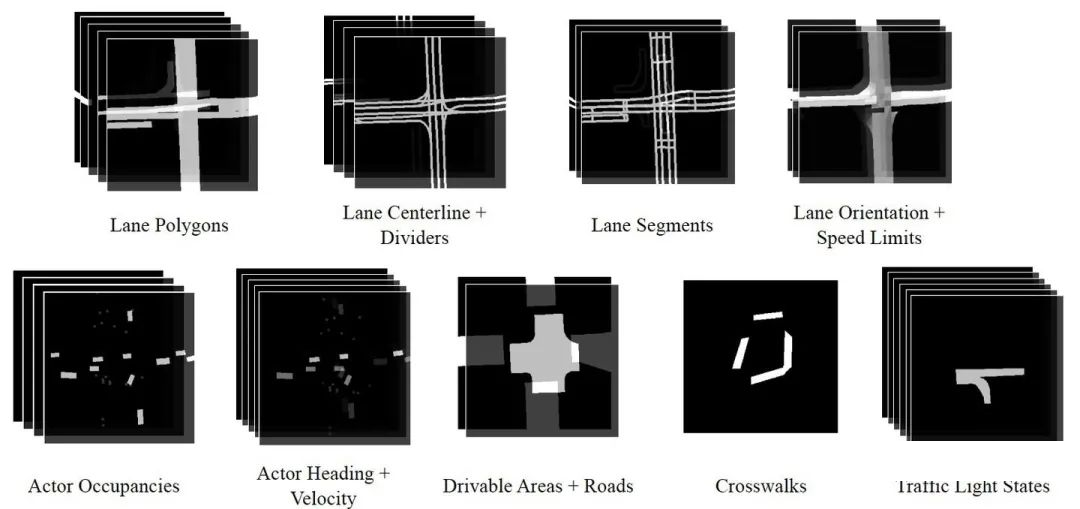
”TrafficSim: Learning to Simulate Realistic Multi-Agent
Behaviors“
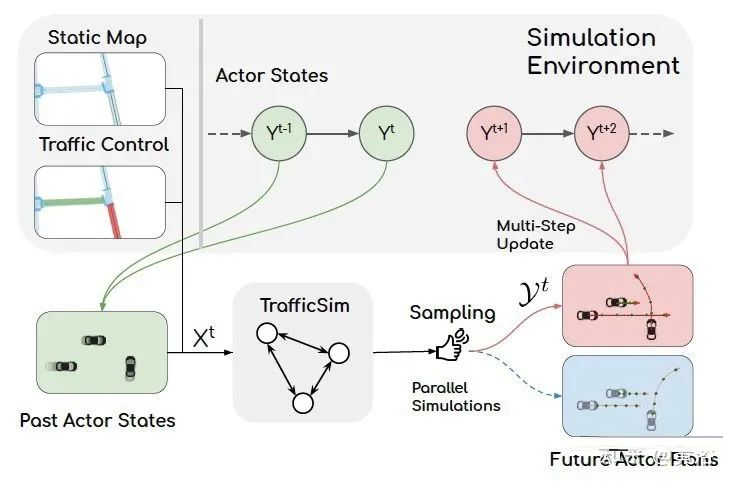
”GeoSim: Realistic Video Simulation via Geometry-Aware
Composition for Self-Driving“

“AdvSim: Generating Safety-Critical Scenarios for
Self-Driving Vehicles“

另外谷歌Waymo最近推出的传感器仿真工作:
”SurfelGAN: Synthesizing Realistic Sensor Data for
Autonomous Driving“
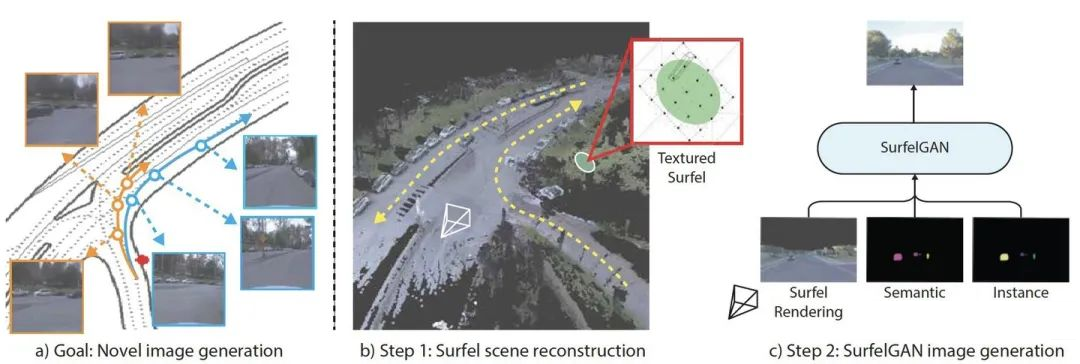
二是封闭场地的实车测试检验。各大车企都有自己的测试基地,现在自动驾驶方面也可使用。其开销远大于仿真系统。
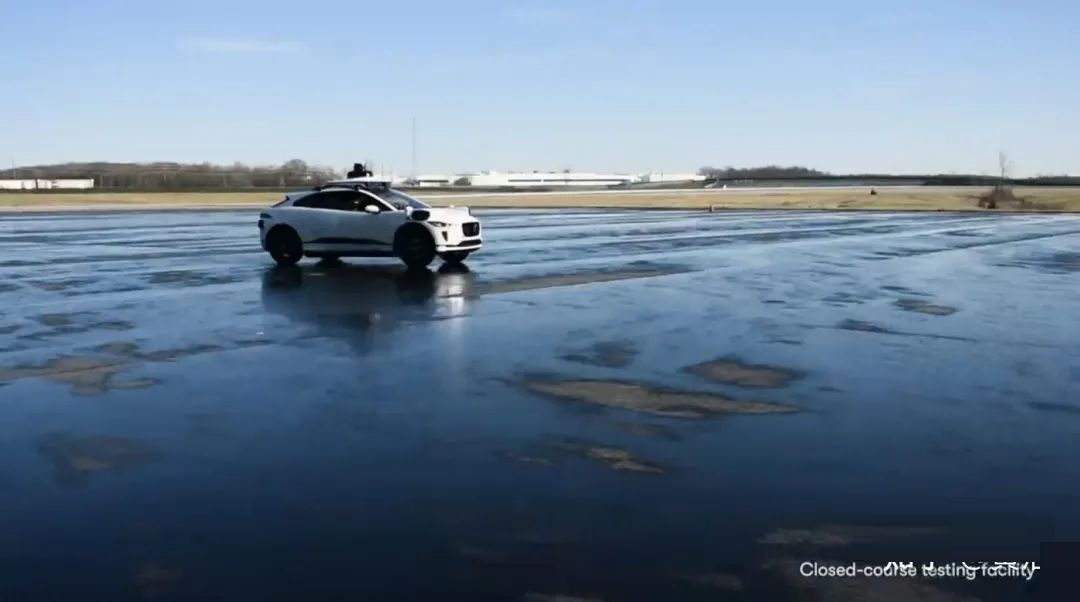
谷歌waymo测试场
三是开放场地的测试检验。现在好多L4级别的自动驾驶,比如无人出租车/无人卡车/无人送货,都在做这种政府批准的实际驾驶实验区测试。这种测试,必要时候安全员的接管或者遥控接管,都是需要花费大量投入的。
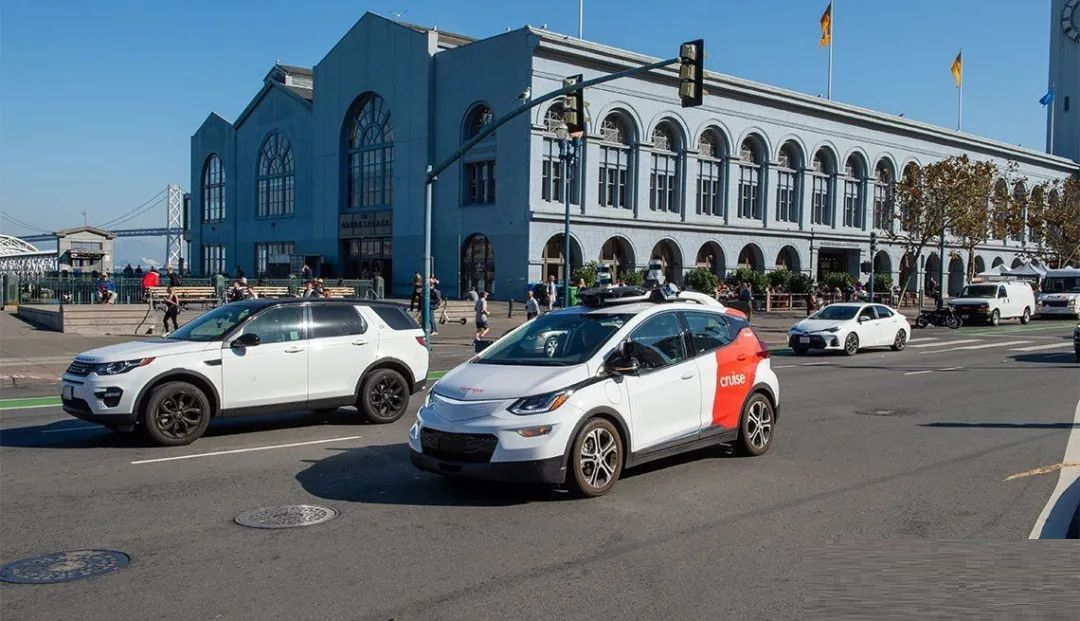
GM的自动驾驶公司Cruise在旧金山测试
最后是用户的测试检验。这个是特斯拉特有的影子模式,以及FSD beta版本测试的志愿者模式。
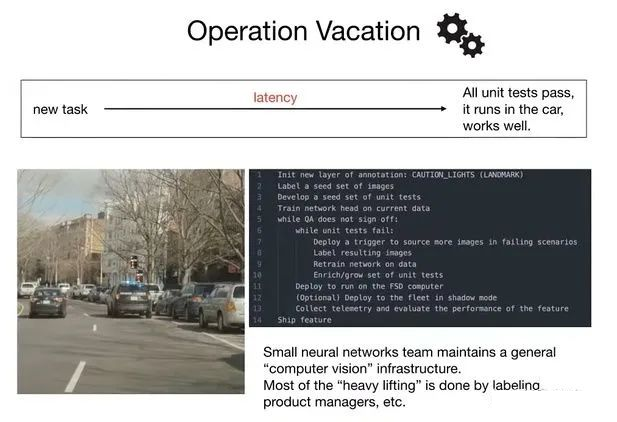
特斯拉的“Operation Vacation”模式
如图是英伟达报告描述的车队级别的模型部署闭环:
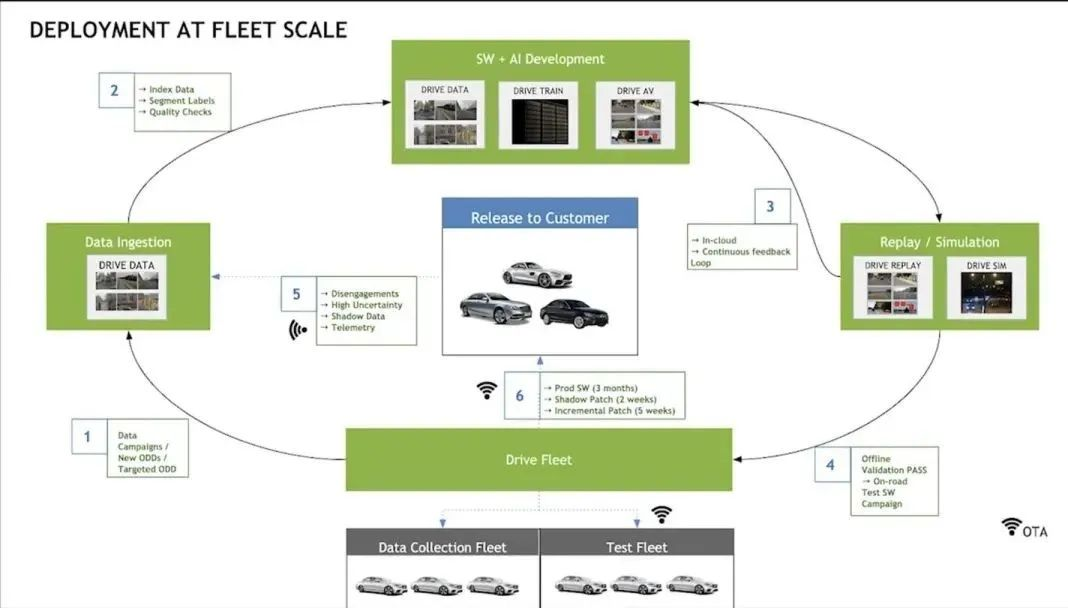
6 相关的机器学习技术
最后,谈一下数据闭环所采用的机器学习技术,其实就是选择什么训练数据和如何迭代更新模型的策略。主要有以下几点:
主动学习
OOD检测和Corner Case检测
数据增强/对抗学习
迁移学习/域自适应
自动机器学习(AutoML )/元学习(学习如何学习)
半监督学习
自监督学习
少样本/ 零样本学习
持续学习/开放世界
下面分别讨论:
1)主动学习
主动学习(active learning)的目标是找到有效的方法从无标记数据池中选择要标记的数据,最大限度地提高准确性。主动学习通常是一个迭代过程,在每次迭代中学习模型,使用一些启发式方法从未标记数据池中选择一组数据进行标记。因此,有必要在每次迭代中为了大子集查询所需标签,这样即使对大小适中的子集,也会产生相关样本。
如图是一个主动学习闭环示意图:在无标注数据中查询、标注所选择数据、添加标注数据到训练集和模型训练。
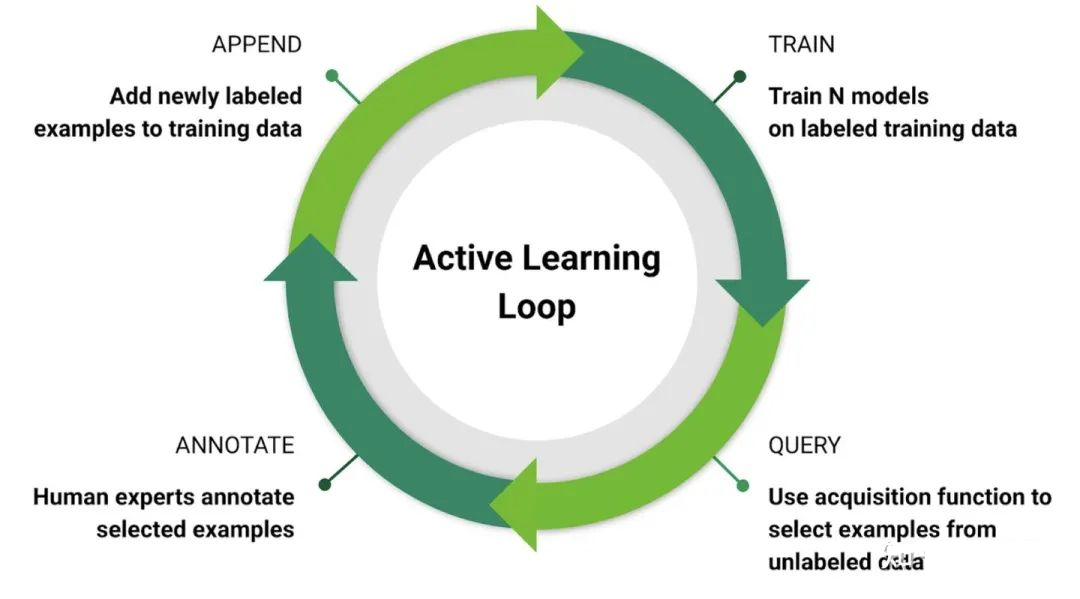
一些方法把标注和无标注数据放在一起,故此采用监督学习和半监督学习进行训练。
贝叶斯主动学习方法通常使用非参数模型(如高斯过程)来估计每个查询的预期进步或一组查询后的预期错误。
基于不确定性主动学习方法尝试使用启发式方法,比如最高熵,和决策边界的几何距离等来寻找困难例子(hard
examples)。
如图是英伟达基于主动学习的挖掘数据方法:
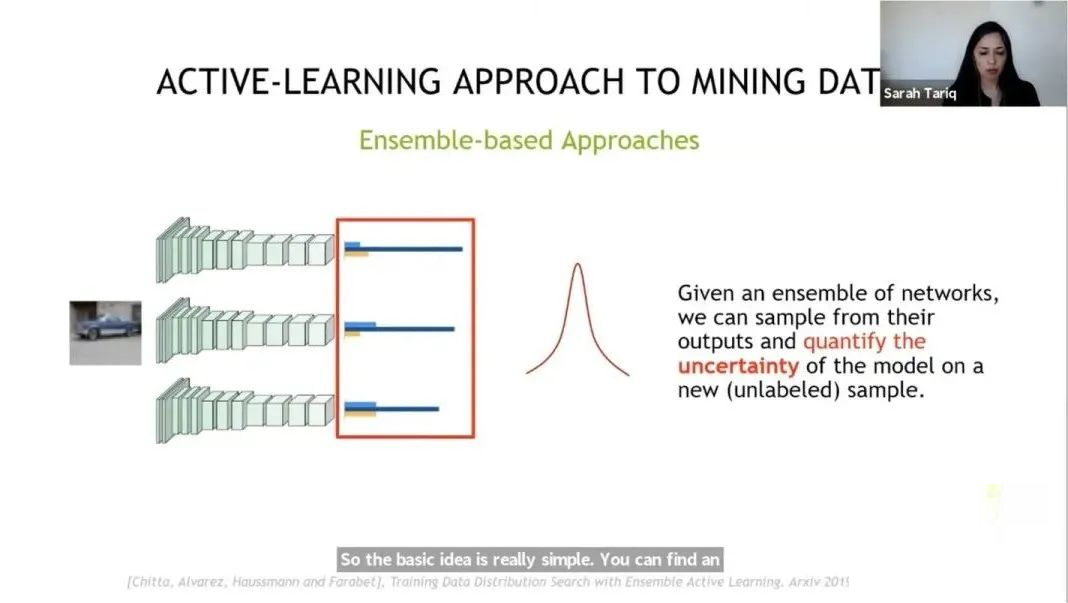
还有其他的主动学习实例方法:
“Deep Active Learning for Efficient Training of a
LiDAR 3D Object Detector“
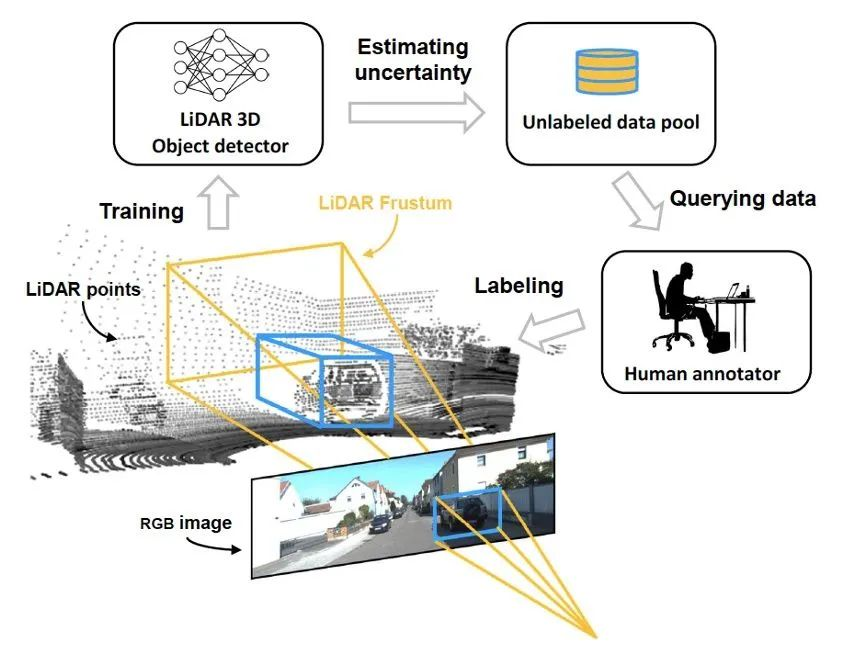
“Consistency-based Active Learning for Object Detection“
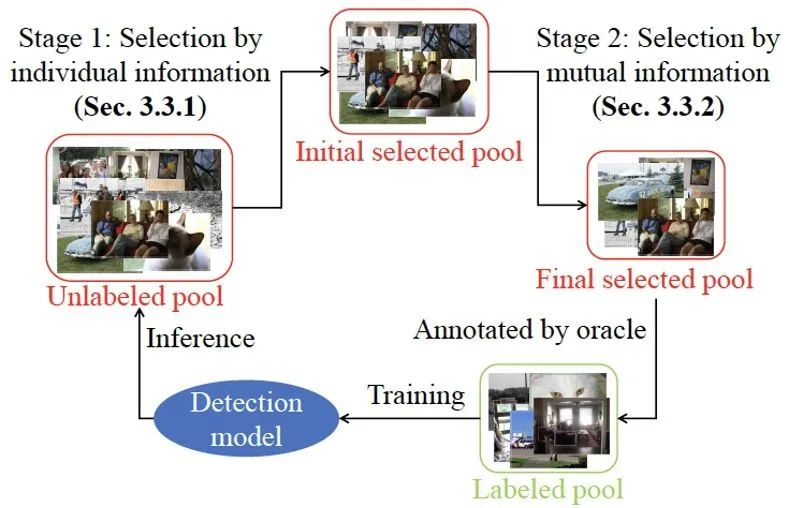
2)OOD检测和Corner Case检测
机器学习模型往往会在out-of-distribution(OOD) 数据上失败。检测OOD是确定不确定性(Uncertainty)的手段,既可以安全报警,也可以发现有价值的数据样本。
不确定性有两种来源:任意(aleatoric)不确定性和认知(epistemic)不确定性。
导致预测不确定性的数据不可减(Irreducible)不确定性,是一种任意不确定性(也称为数据不确定性)。任意不确定性有两种类型:同方差(homo-scedastic)和异方差(hetero-scedastic)。
另一类不确定性是由于知识和数据不适当造成的认知不确定性(也称为知识/模型不确定性)。
最常用的不确定性估计方法是贝叶斯近似(Bayesian approximation)法和集成学习(ensemble
learning)法。
一类 OOD 识别方法基于贝叶斯神经网络推理,包括基于 dropout 的变分推理(variational
inference)、马尔可夫链蒙特卡罗 (MCMC) 和蒙特卡罗 dropout等。
另一类OOD识别方法包括 (1) 辅助损失或NN 架构修改等训练方法,以及 (2) 事后统计(post
hoc statistics)方法。
数据样本中有偏离正常的意外情况,即所谓的corner case。可靠地检测此类corner case,在开发过程中,在线和离线应用都是必要的。
在线应用可以用作安全监控和警告系统,在corner case情况发生时进行识别。离线应用将corner
case检测器应用于大量收集的数据,选择合适的训练和相关测试数据。
最近的一些实例工作有:
“Towards Corner Case Detection for Autonomous Driving“
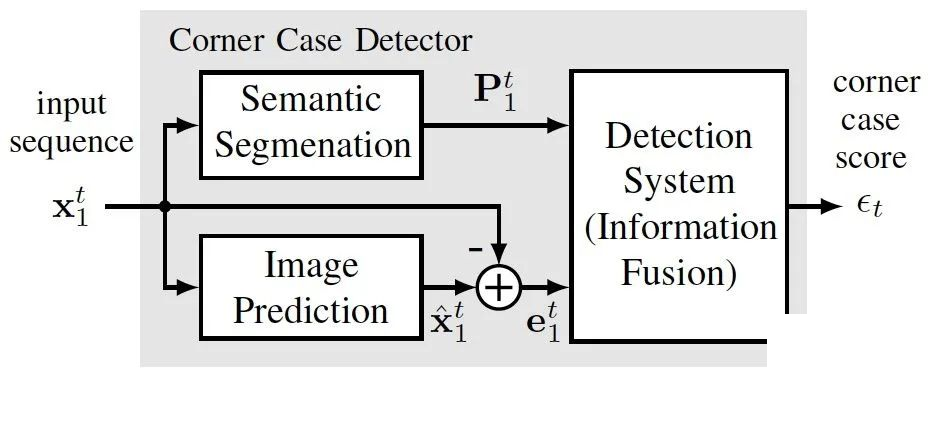
“Out-of-Distribution Detection for Automotive Perception“
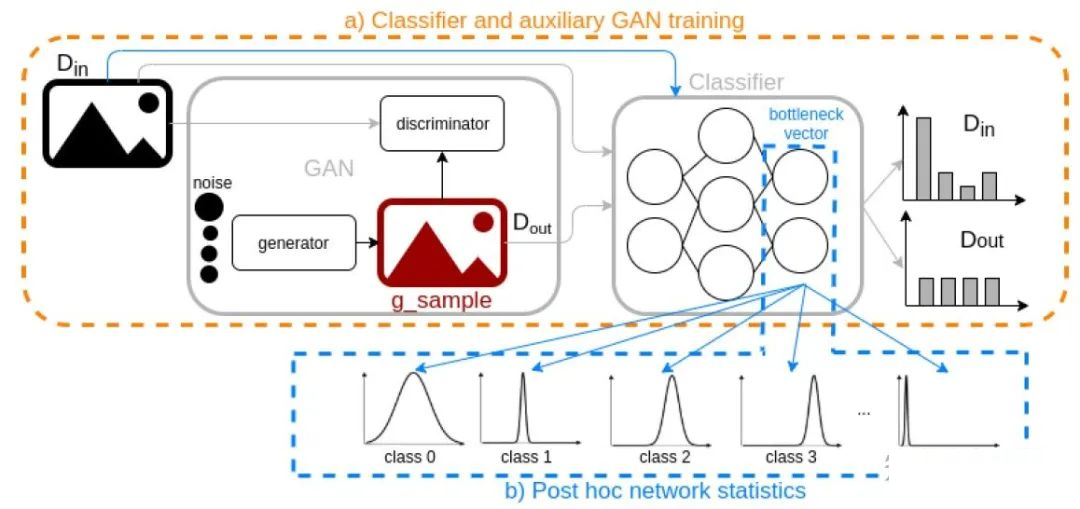
“Corner Cases for Visual Perception in Automated
Driving: Some Guidance on Detection Approaches“
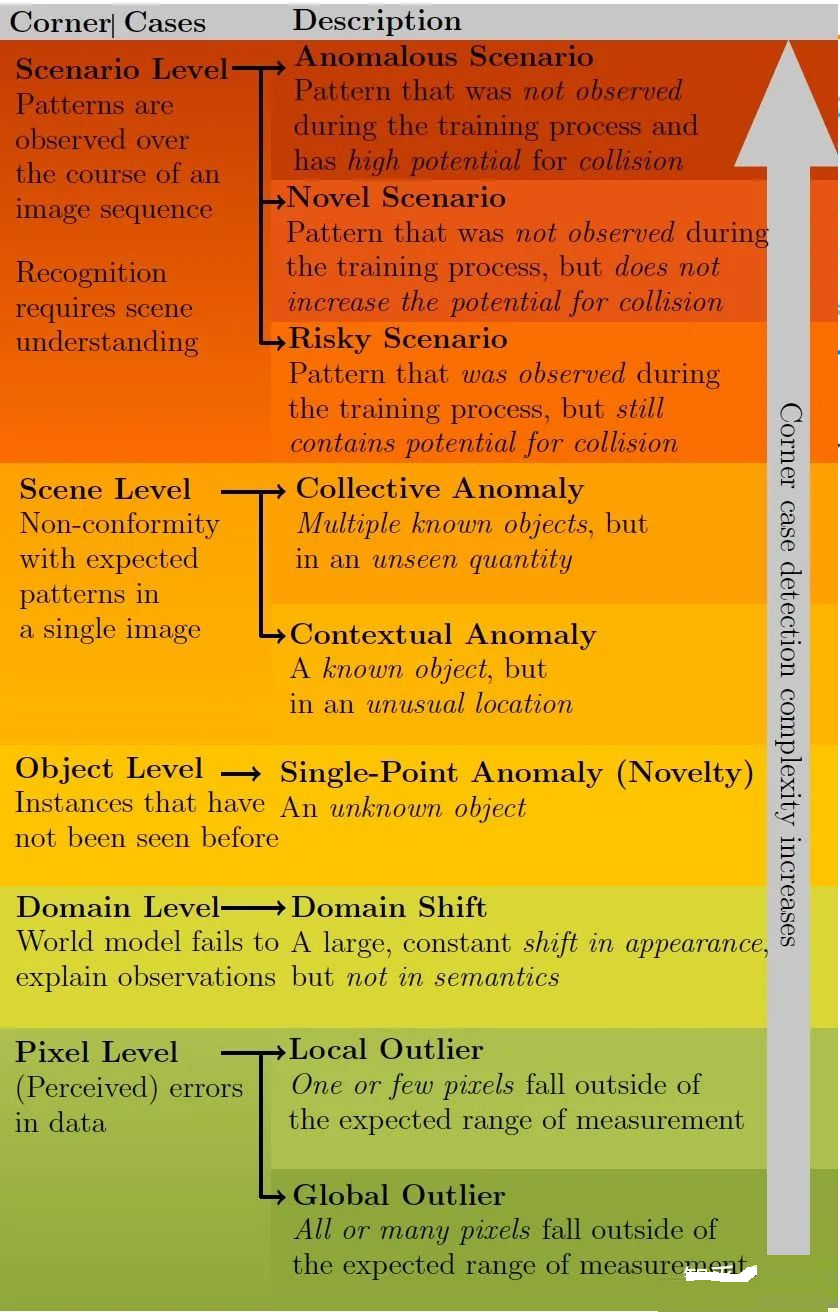
3)数据增强/对抗学习
过拟合(Overfitting)是指当机器学习模型学习高方差的函数完美地对训练数据建模时出现的现象。数据增强(Data
Augmentation)增强训练数据集的大小和质量,克服过拟合,从而构建更好的机器学习模型。
图像数据增强算法包括几何变换、色彩空间增强、内核过滤器、混合图像、随机擦除、特征空间增强、对抗训练(adversarial
training)、生成对抗网络(generative adversarial networks,GAN)、神经风格迁移(neural
style transfer)和元学习(meta-learning)。
激光雷达点云数据的增强方法还有特别的一些:全局变换(旋转、平移、尺度化)、局部变换(旋转、平移、尺度化)和3-D滤波。
对抗性训练可以成为寻找增强方向的有效方法。通过限制对抗网络(adversarial network)可用的增强和畸变变换集,通过学习得到导致错误的增强方式。这些增强对于加强机器学习模型中的弱点很有价值。
值得一提的是,CycleGAN 引入了一个额外的 Cycle-Consistency 损失函数,稳定
GAN 训练,应用于图像到图像转换(image-to-image translation)。实际上CycleGAN
学习从一个图像域转换到另一个域。
机器学习模型错误背后的一个常见原因是一种称为数据集偏差或域漂移(dataset bias / domain
shift)的现象。域适应方法试图减轻域漂移的有害作用。对抗训练方法引入到域适应,比如对抗鉴别域适应方法(Adversarial
Discriminative Domain Adaptation,ADDA)。
最近出现的一些新实例方法:
“AutoAugment: Learning Augmentation Strategies from
Data“
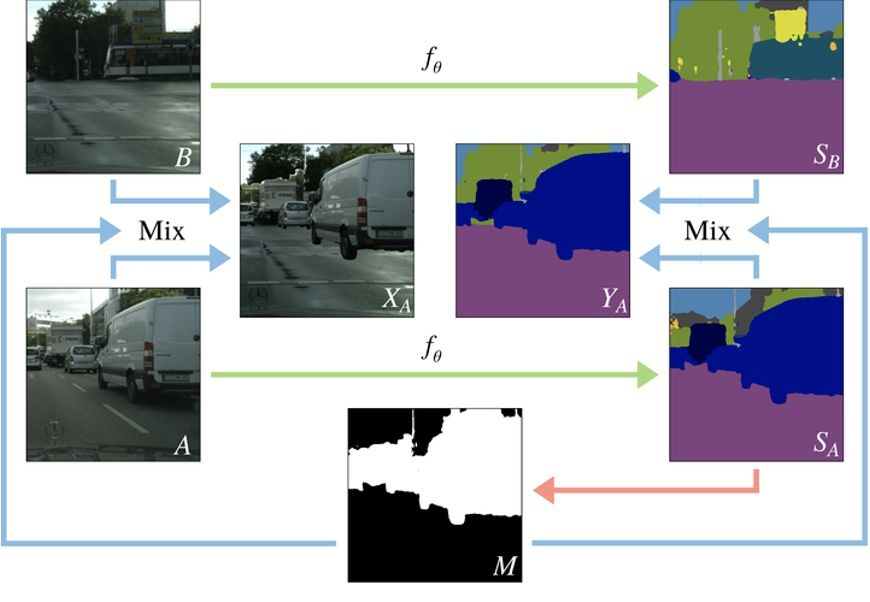
“Classmix: Segmentation-based Data Augmentation For
Semi-supervised Learning“
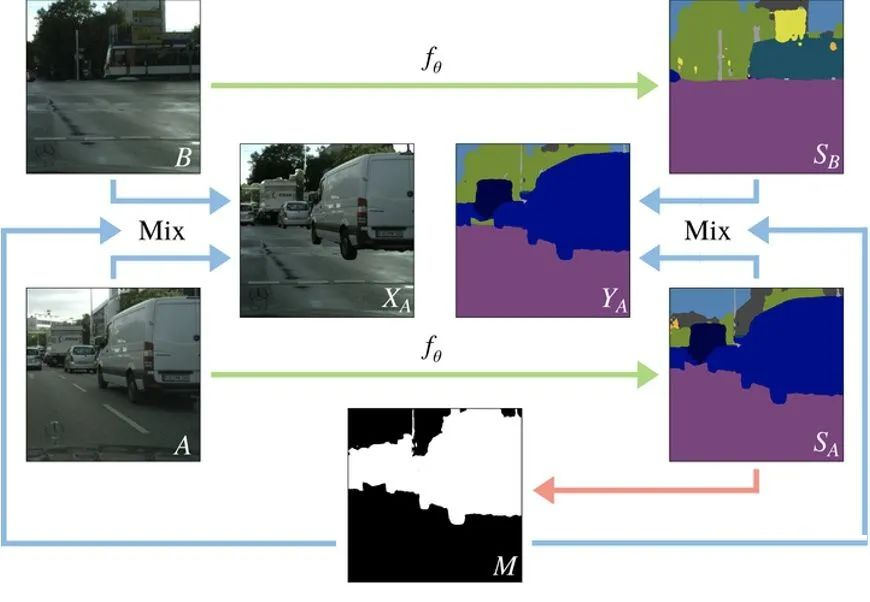
“Data Augmentation for Object Detection via Differentiable
Neural Rendering“
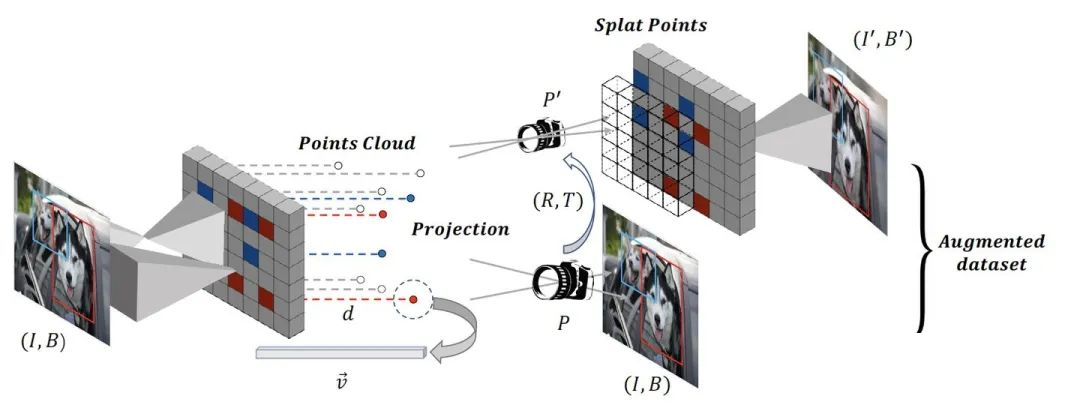
“LiDAR-Aug: A General Rendering-based Augmentation
Framework for 3D Object Detection“
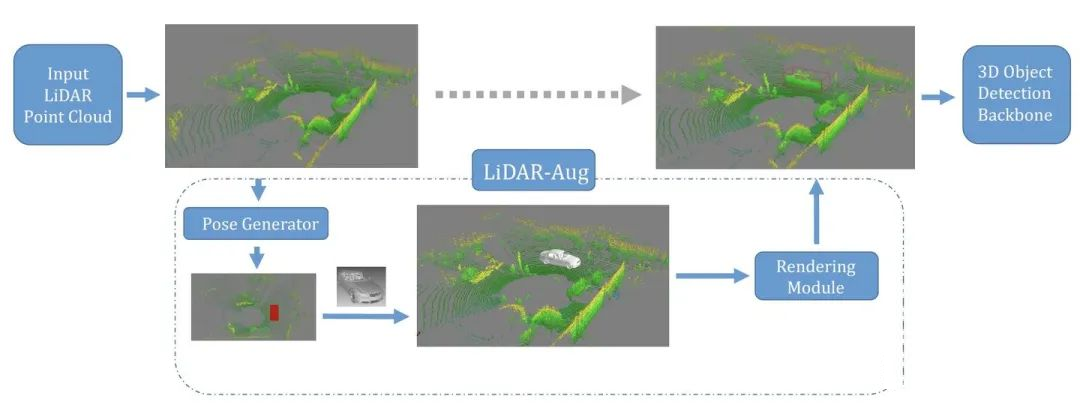
“Adaptive Object Detection with Dual Multi-Label
Prediction“
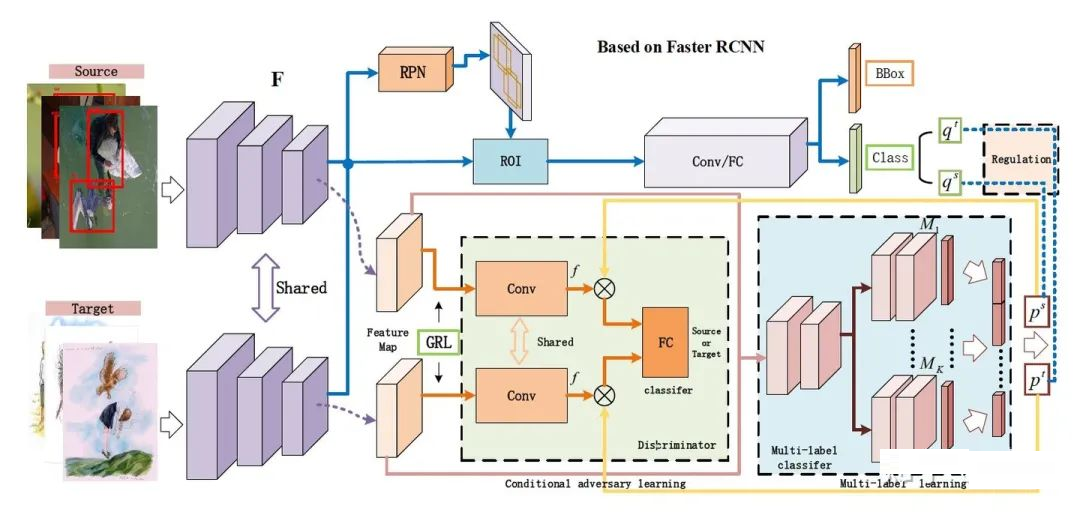
“Taking A Closer Look at Domain Shift: Category-level
Adversaries for Semantics Consistent Domain Adaptation“
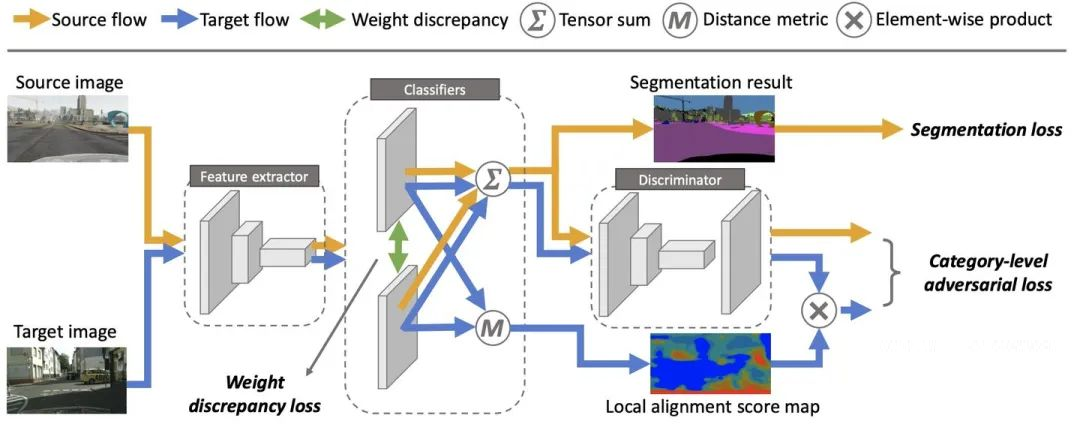
4)迁移学习/域适应
迁移学习(transfer learning,TL)不需要训练数据和测试数据是独立同分布(independent
and identically distributed,i.i.d),目标域的模型不需要从头开始训练,可以减少目标域训练数据和时间的需求。
深度学习的迁移技术基本分为两种类型,即非对抗性的(传统)和对抗性的。
域适应 (domain adaptation,DA) 是TL的一种特殊情况,利用一个或多个相关源域(source
domains)的标记数据在目标域(target domain)执行新任务。
DA方法分为两类:基于实例的和基于特征的。
最近出现的一些新实例方法:
“Multi-Target Domain Adaptation via Unsupervised
Domain Classification for Weather Invariant Object
Detection“
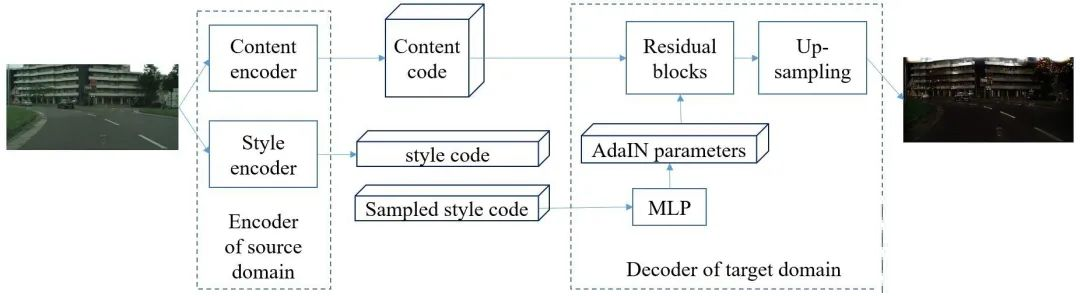
“Uncertainty-Aware Consistency Regularization for
Cross-Domain Semantic Segmentation“
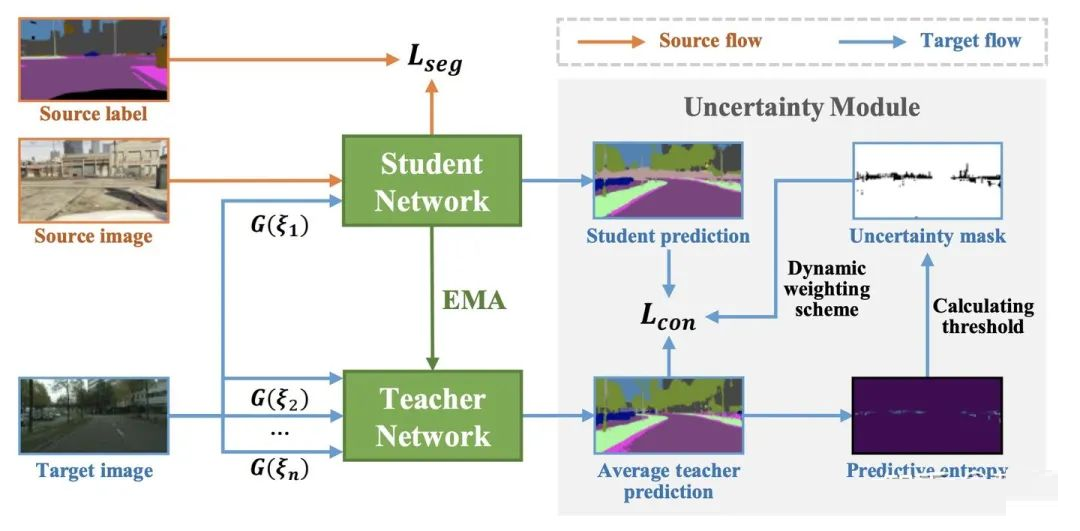
“SF-UDA3D: Source-Free Unsupervised Domain Adaptation
for LiDAR-Based 3D Object Detection“
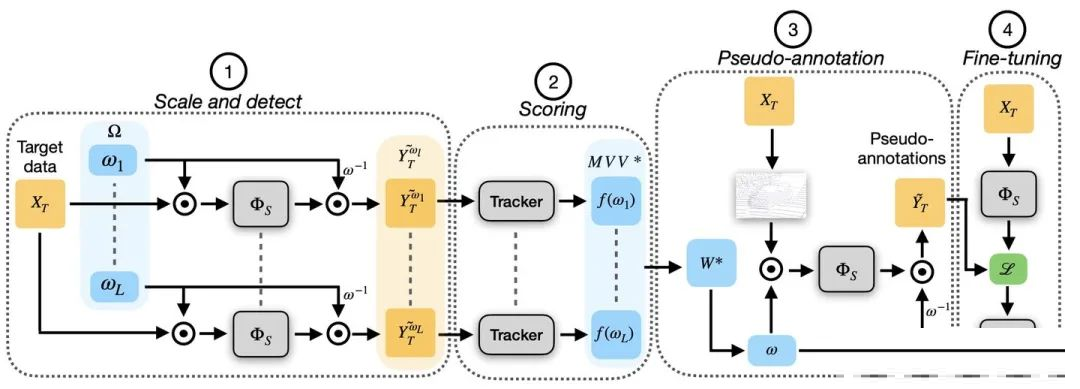
“LiDARNet: A Boundary-Aware Domain Adaptation Model
for Point Cloud Semantic Segmentation“
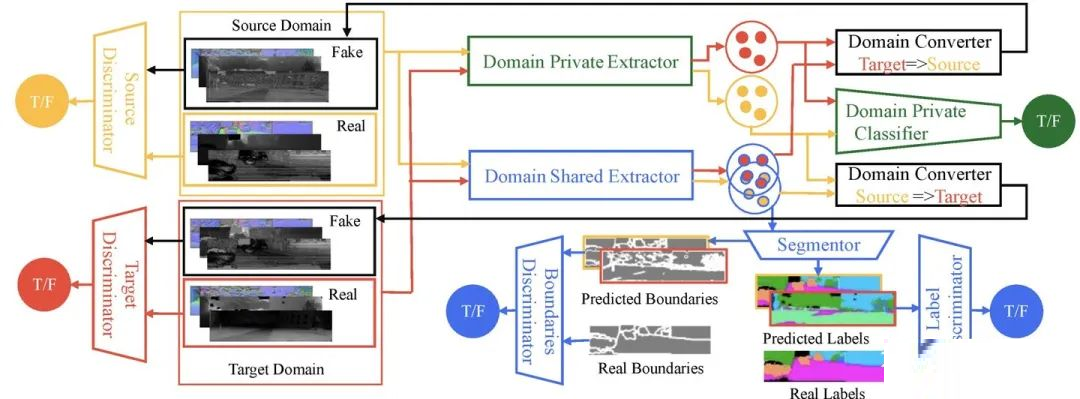
5)自动机器学习(AutoML)/元学习(学习如何学习)
一个机器学习建模的工程还有几个方面需要人工干预和可解释性,即机器学习落地流水线的两个主要组件:预-建模和后-建模(如图)。
预-建模影响算法选择和超参数优化过程的结果。预-建模步骤包括多个步骤,包括数据理解、数据准备和数据验证。
后-建模模块涵盖了其他重要方面,包括机器学习模型的管理和部署。
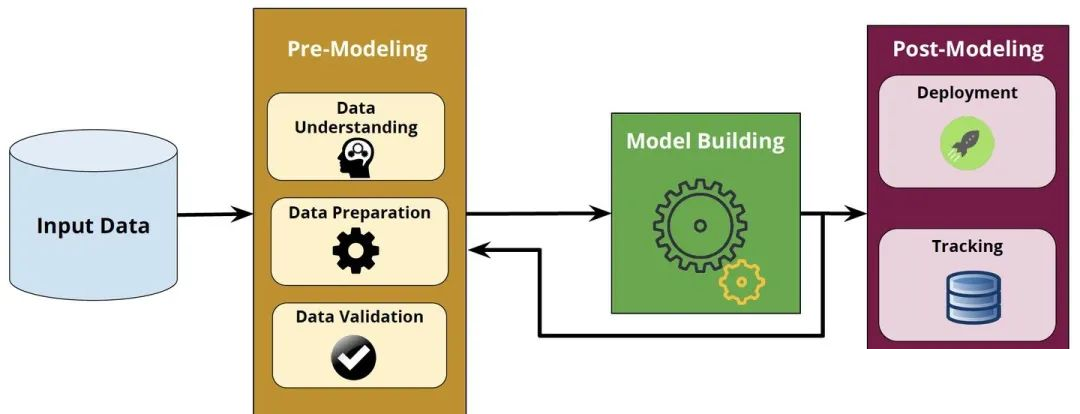
为了降低这些繁重的开发成本,出现了自动化整个机器学习流水线的新概念,即开发自动机器学习(automated
machine learning,AutoML) 方法。AutoML 旨在减少对数据科学家的需求,并使领域专家能够自动构建机器学习应用程序,而无需太多统计和机器学习知识。
值得特别一提的是谷歌方法“神经架构搜索”(Neural Architecture Search,NAS),其目标是通过在预定义搜索空间中选择和组合不同的基本组件来生成稳健且性能良好的神经网络架构。
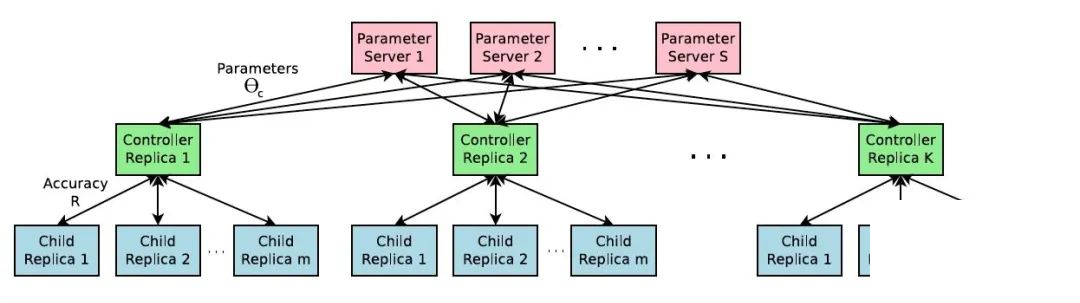
NAS的分布式训练实现
NAS的总结从两个角度了解:模型结构类型和采用超参数优化(hyperparameter optimization,HPO)的模型结构设计。最广泛使用的
HPO 方法利用强化学习 (RL)、基于进化的算法 (EA)、梯度下降 (GD) 和贝叶斯优化 (BO)方法。
如图是AutoML在机器学习平台的应用实例:
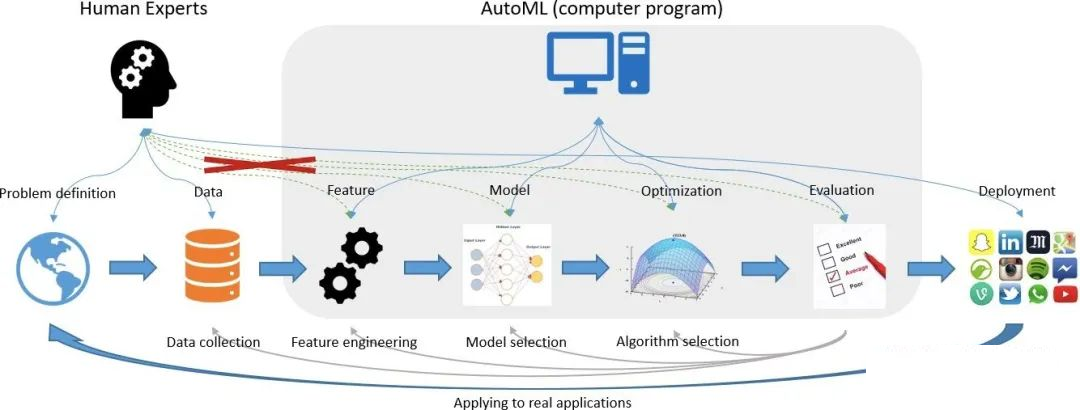
注:在谷歌云、微软云Azure和亚马逊云AWS都支持AutoML。
深度学习(DL)专注于样本内预测,元学习(meta learning)关注样本外预测的模型适应问题。元学习作为附加在原始
DL 模型的泛化部分。
元学习寻求模型适应与训练任务大不相同的未见过的任务(unseen tasks)。元强化学习 (meta-RL)
考虑代理与不断变化的环境之间的交互过程。元模仿学习 (Meta-IL) 将过去类似的经验应用于只有稀疏奖励的新任务。
元学习与 AutoML 密切相关,二者有相同的研究目标,即学习工具和学习问题。现有的元学习技术根据在
AutoML 的应用可分为三类:
1)用于配置评估(对于评估者);
2)用于配置生成(用于优化器);
3) 用于动态配置的自适应。
元学习促进配置生成,例如,针对特定学习问题的配置、生成或选择配置策略或细化搜索空间。元学习检测概念漂移(concept
drift)并动态调整学习工具实现自动化机器学习(AutoML)过程。
6)半监督学习
半监督学习(semi-supervised learning)是利用未标记数据生成具有可训练模型参数的预测函数,目标是比用标记数据获得的预测函数更准确。由于混合监督和无监督方法,半监督学习的损失函数可以具有多种形状。一种常见的方法是添加一个监督学习的损失项和一个无监督学习的损失项。
已经有一些经典的半监督学习方法:
“Pseudo-label: The simple and efficient semi-supervised
learning method for deep neural networks”
“Mean teachers are better role models: Weight-averaged
consistency targets improve semi-supervised deep learning
results“
“Self-training with Noisy Student improves ImageNet
classification“
最近出现一些新实例方法:
“Unbiased Teacher for Semi-Supervised Object Detection“
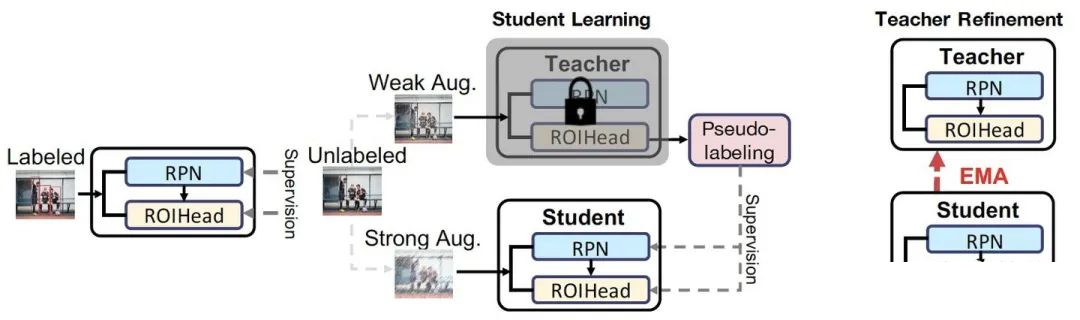
“Pseudoseg: Designing Pseudo Labels For Semantic
Segmentation“
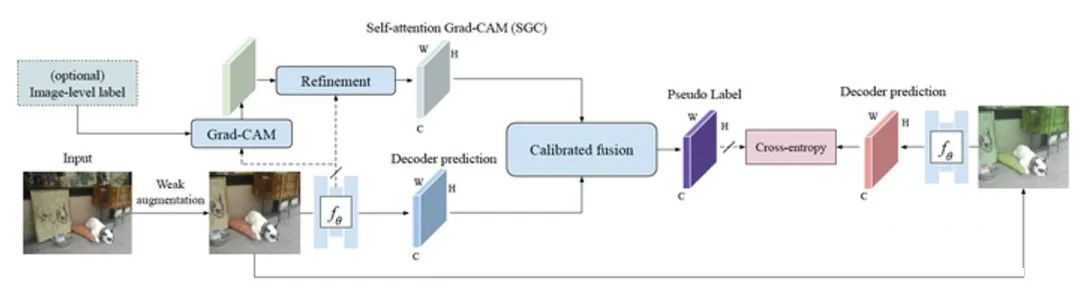
“Semantic Segmentation of 3D LiDAR Data in Dynamic
Scene Using Semi-supervised Learning“
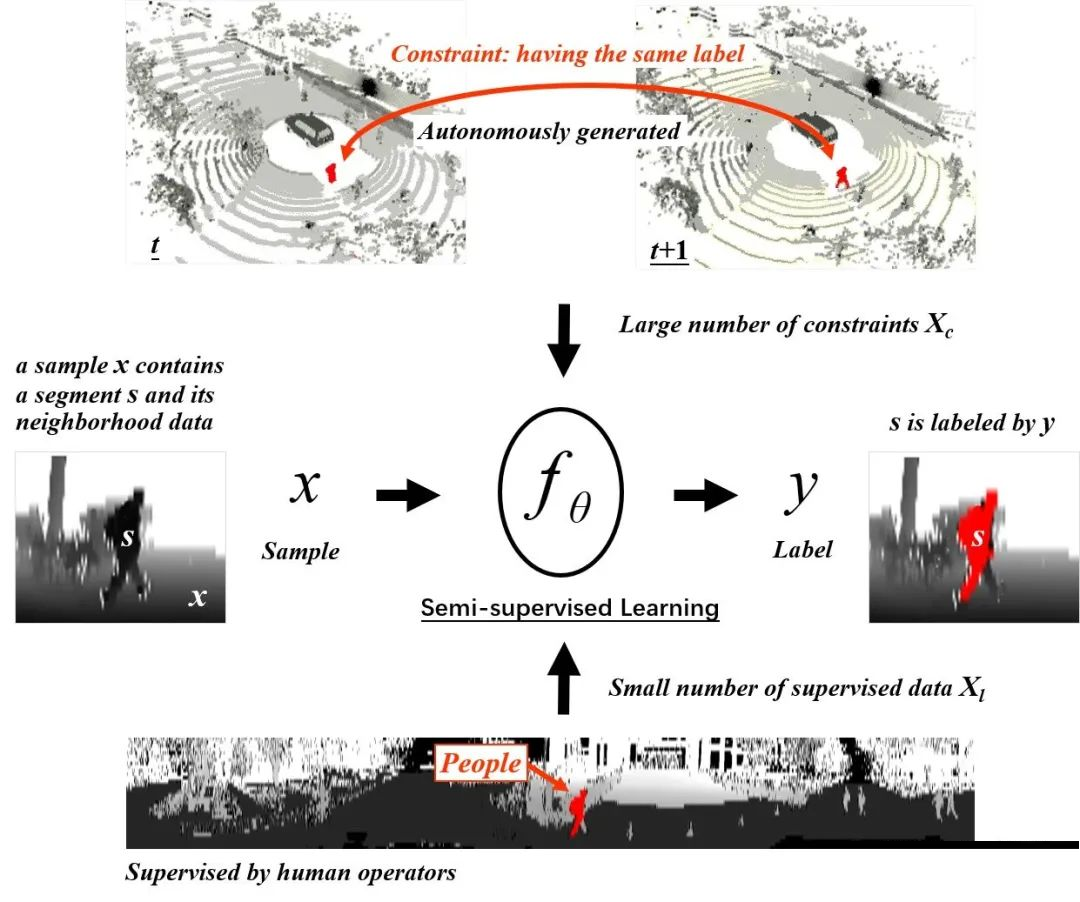
“ST3D: Self-training for Unsupervised Domain Adaptation
on 3D Object Detection“
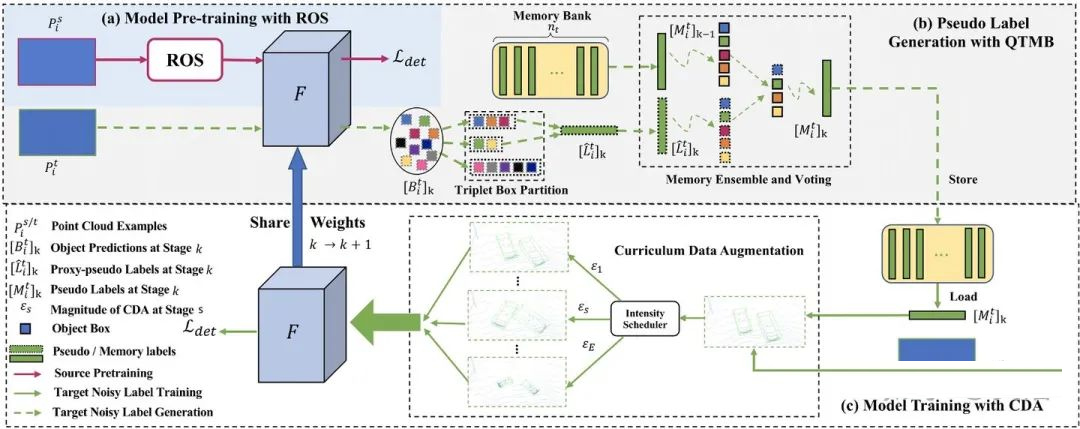
“3DIoUMatch: Leveraging IoU Prediction for Semi-Supervised
3D Object Detection“
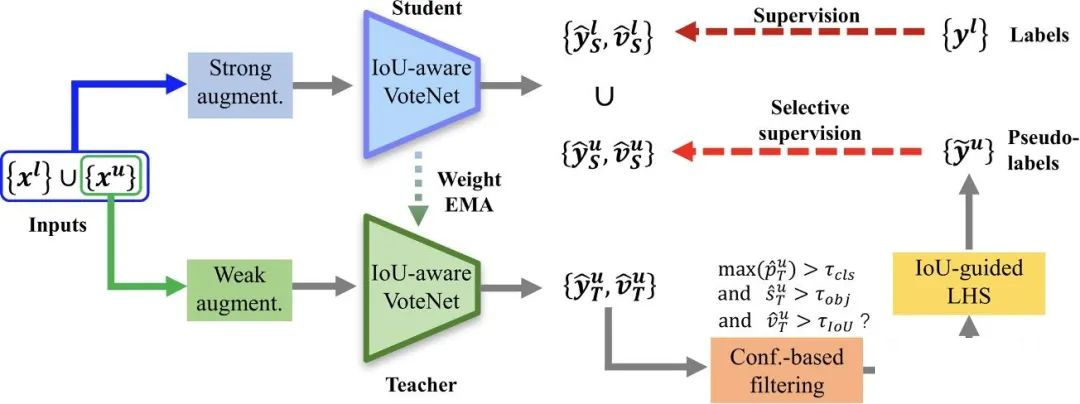
7)自监督学习
自监督学习(self supervised learning)算是无监督学习的一个分支,其目的是恢复,而不是发现。自监督学习基本分为:生成(generative)类,
对比(contrastive)类和生成-对比(generative-contrastive)混合类,即对抗(adversarial)类。
自监督使用借口任务(pretext task)来学习未标记数据的表示。借口任务是无监督的,但学习的表示通常不能直接给下游任务(downstream
task),必须进行微调。因此,自监督学习可以被解释为一种无监督、半监督或自定义策略。下游任务的性能用于评估学习特征的质量。
一些著名的自监督学习方法有:
“SimCLR-A Simple framework for contrastive learning
of visual representations“
“Momentum Contrast for Unsupervised Visual Representation
Learning“
“Bootstrap Your Own Latent: A New Approach to Self-Supervised
Learning“
“Deep Clustering for Unsupervised Learning of Visual
Features“
“Unsupervised Learning of Visual Features by Contrasting
Cluster Assignments“
注意最近的一些新方法:
“DetCo: Unsupervised Contrastive Learning for Object
Detection“
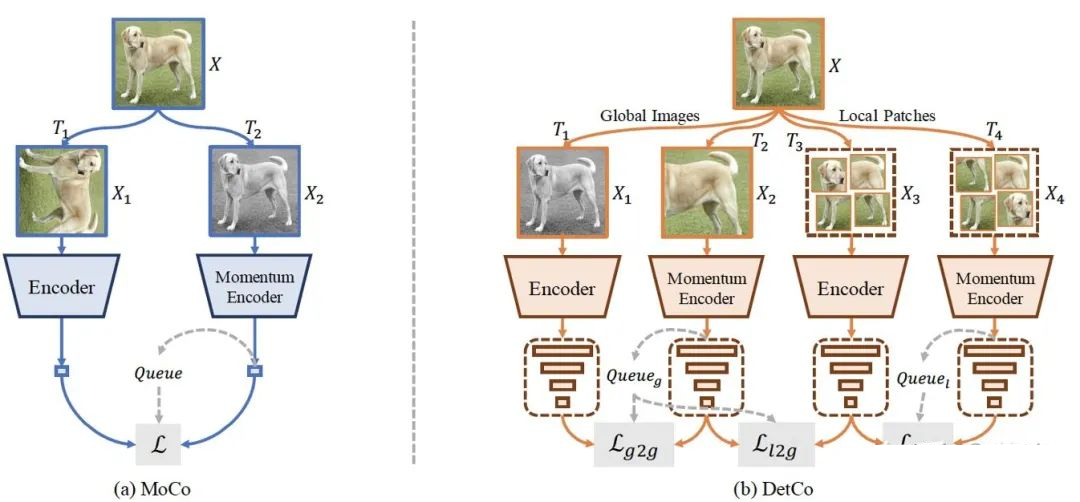
“PointContrast: Unsupervised Pre-training for 3D
Point Cloud Understanding“
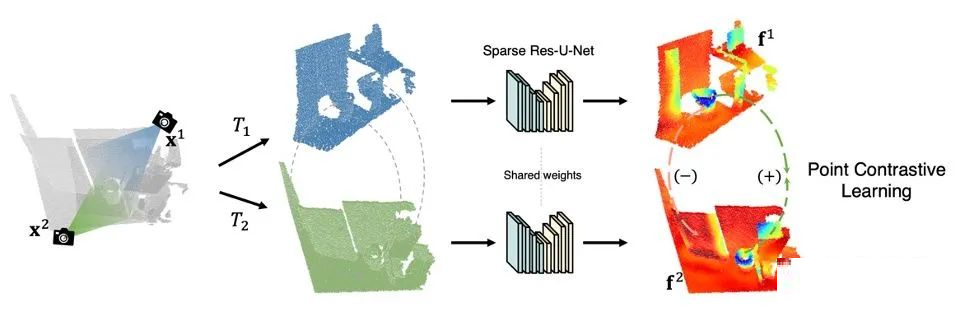
“MonoRUn: Monocular 3D Object Detection by Reconstruction
and Uncertainty Propagation“
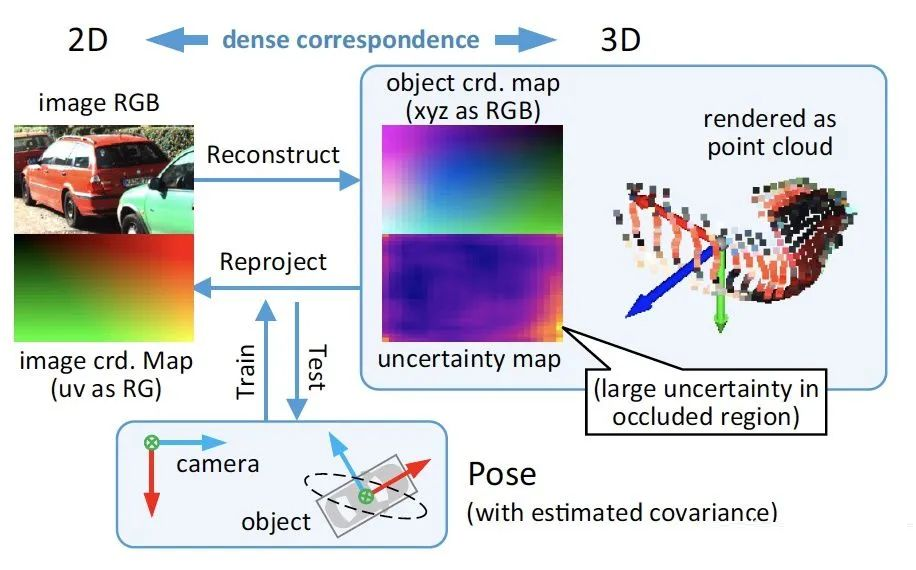
“Weakly Supervised Semantic Point Cloud Segmentation:
Towards 10x Fewer Labels“
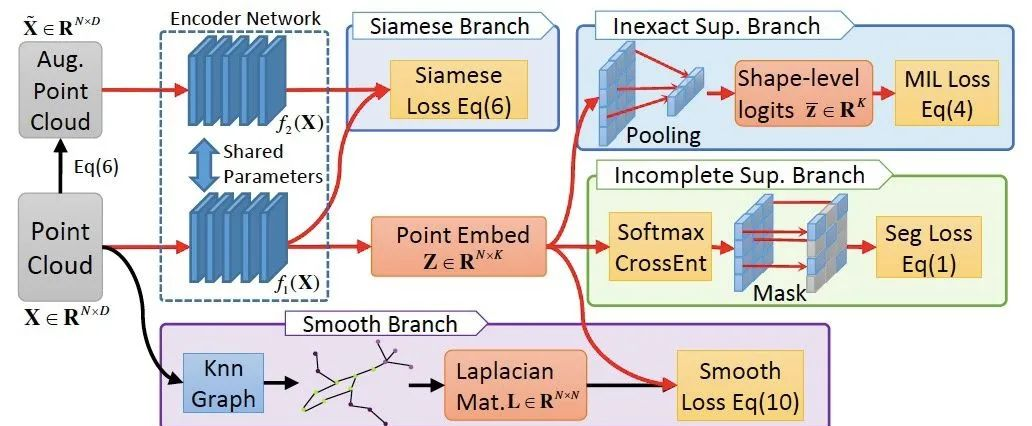
8)少样本/零样本学习
零样本学习(Zero-shot learning,ZSL)旨在识别在训练期间可能未见过实例的目标。虽然大多数ZSL方法都使用判别性损失(discriminative
losses)进行学习,但少数生成模型(generative models)将每个类别表示为概率分布。
对于未见类(unseen classes),ZSL除了无法访问其视觉或辅助信息的inductive设置之外,transductive方法无需访问标签信息,直接用已见类(seen
classes)和未见类一起的视觉或语义信息。
ZSL属于迁移学习(TL),源特征空间为训练实例,目标特征空间为测试实例,二者特征空间一样。但对于已见类和未见类,标签空间是不同的。
为了从有限的监督信息中学习,一个新的机器学习方向称为少样本学习 (Few-Shot Learning
,FSL)。基于如何使用先验知识,FSL可分为三个类:1)用数据先验知识来增强监督经验,2)通过模型先验知识约束假设空间,和3)用算法先验知识改变假设空间中最佳参数的搜索方式。
FSL 可以是监督学习、半监督学习和强化学习(RL),取决于除了有限的监督信息之外还有哪些数据可用。许多
FSL 方法是元学习(meta learning)方法,以此作为先验知识。
最近的一些实例方法:
“Don’t Even Look Once: Synthesizing Features for
Zero-Shot Detection“
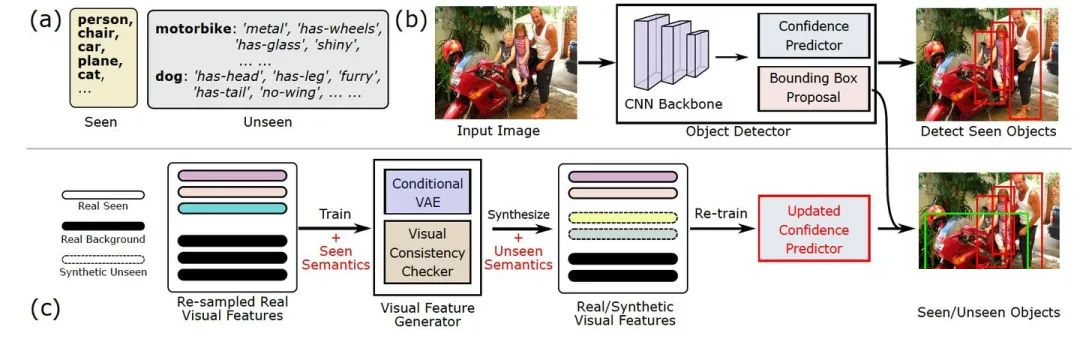
“Zero-Shot Semantic Segmentation“
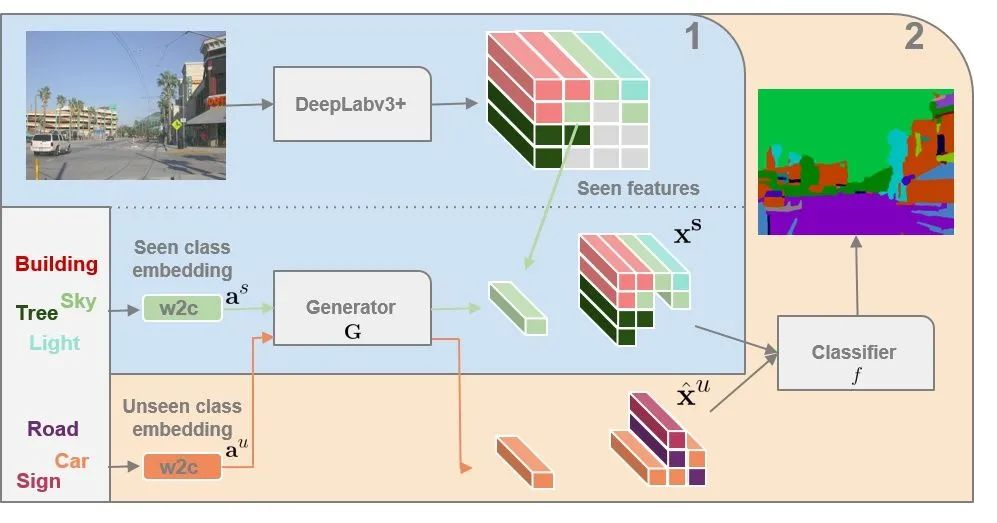
“Zero-Shot Learning on 3D Point Cloud Objects and
Beyond“
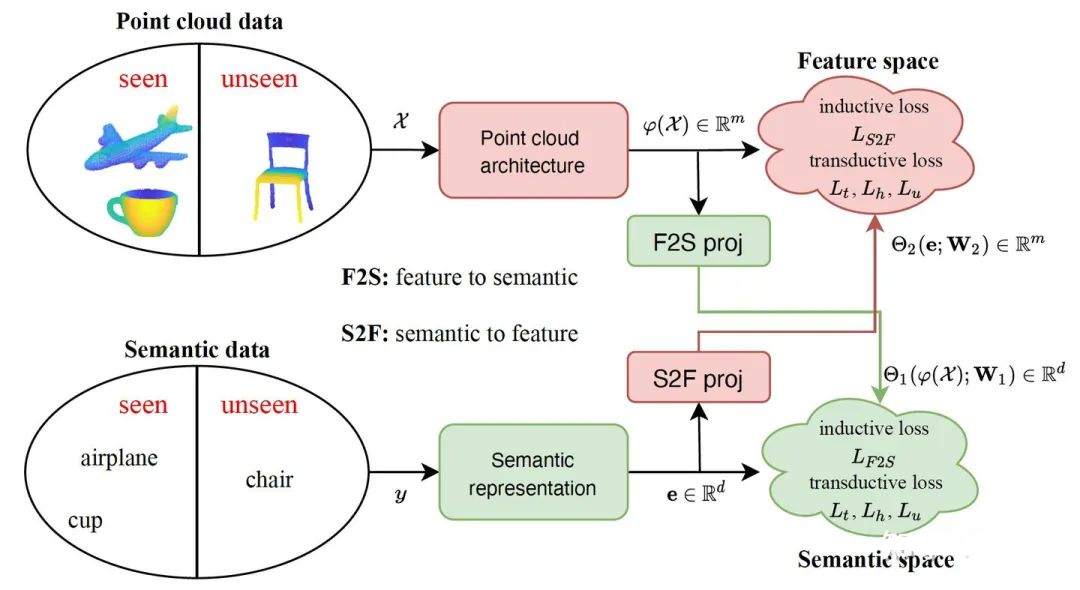
“Few-Shot Object Detection and Viewpoint Estimation
for Objects in the Wild“
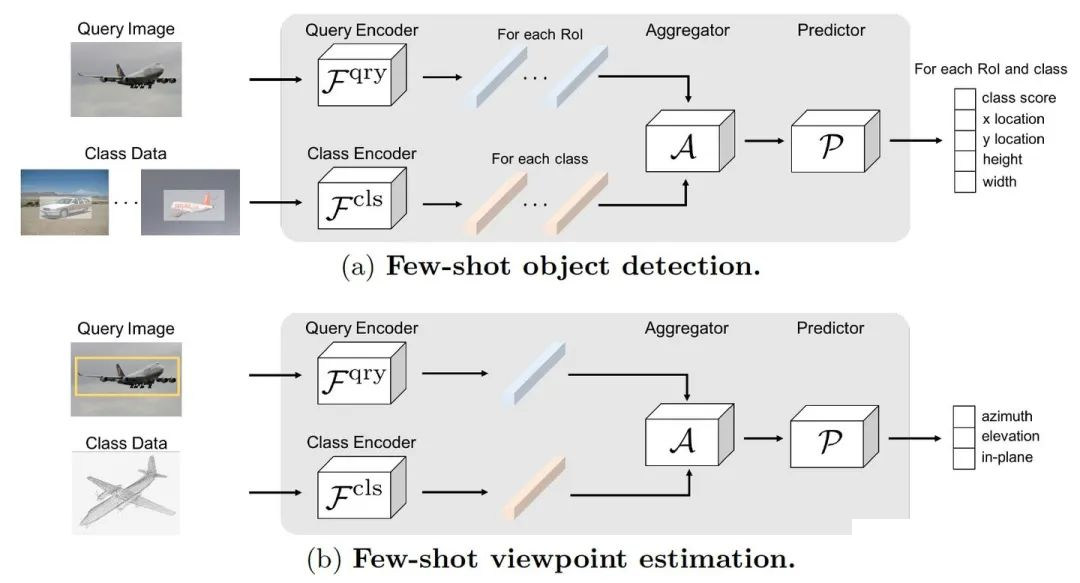
“Self-Supervised Few-Shot Learning on Point Clouds“
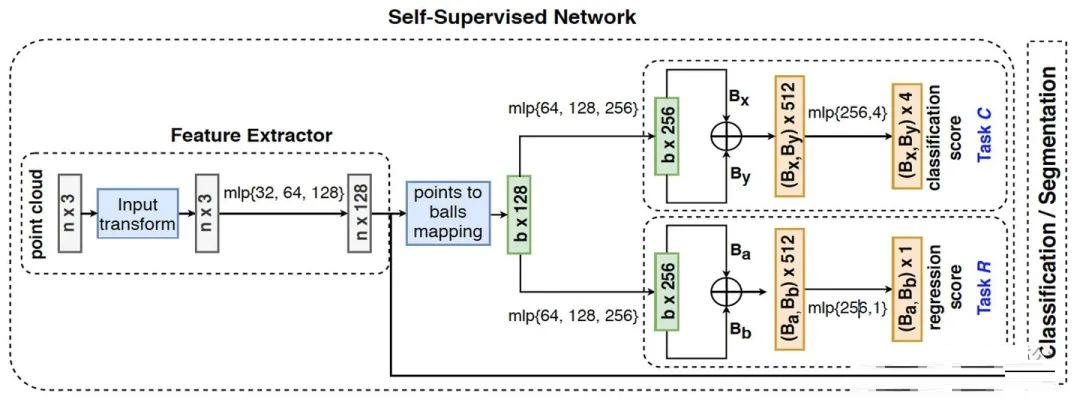
“Few-shot 3D Point Cloud Semantic Segmentation“
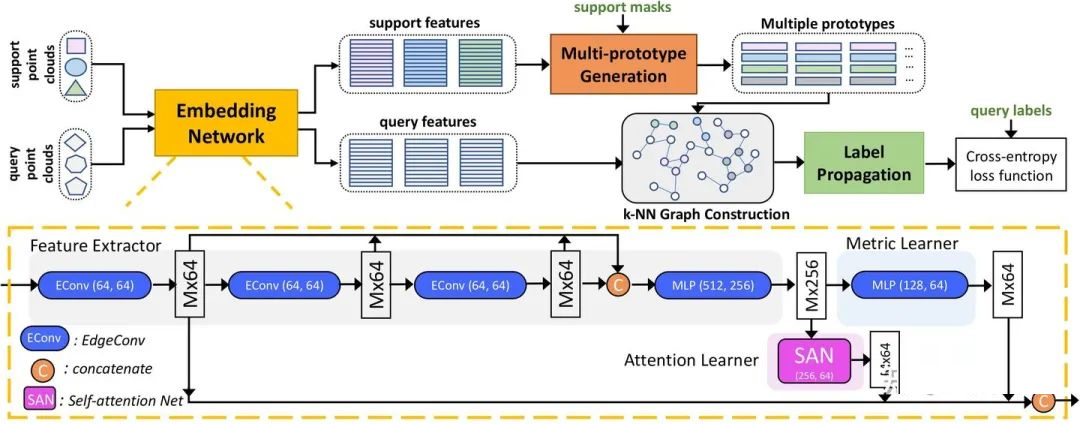
9)持续学习/开放世界
持续学习( continual learning)可以不断积累不同任务得到的知识,而无需从头开始重新训练。其困难是如何克服灾难遗忘(catastrophic
forgetting)。
如图是持续学习的方法分类:经验重放(ER)、正则化和参数孤立三个方向。
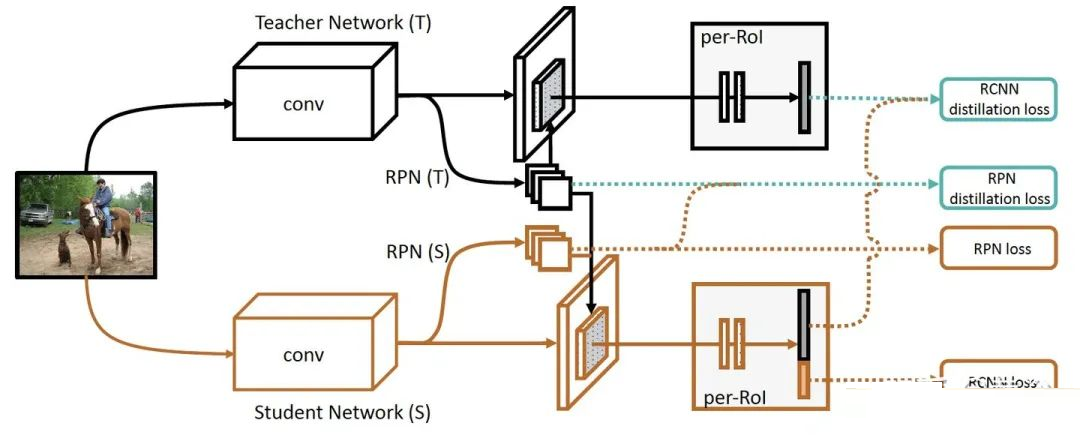
开放集识别(Open set recognition,OSR),是在训练时存在不完整的世界知识,在测试中可以将未知类提交给算法,要求分类器不仅要准确地对所见类进行分类,还要有效处理未见类。开放世界学习(Open
world learning)可以看作是持续学习的一个子任务。
以下给出最近的一些实例方法:
“Lifelong Object Detection“
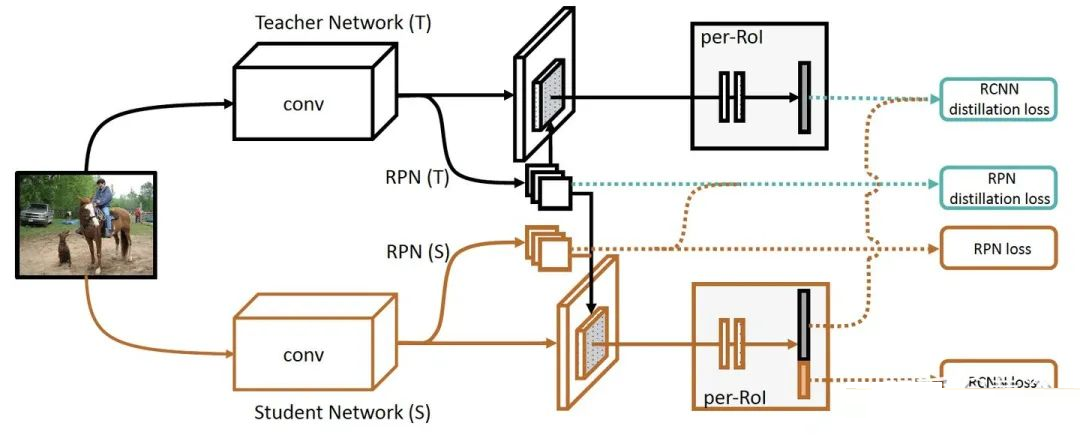
“Incremental Few-Shot Object Detection“
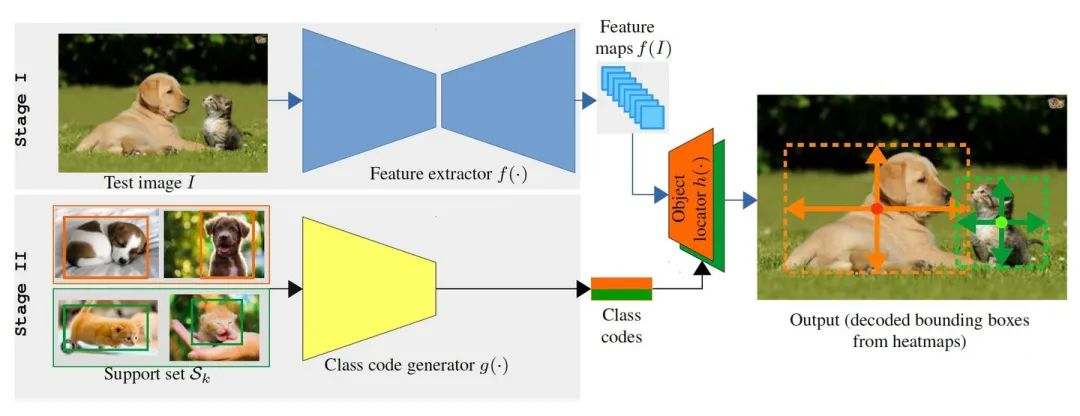
“Towards Open World Object Detection“
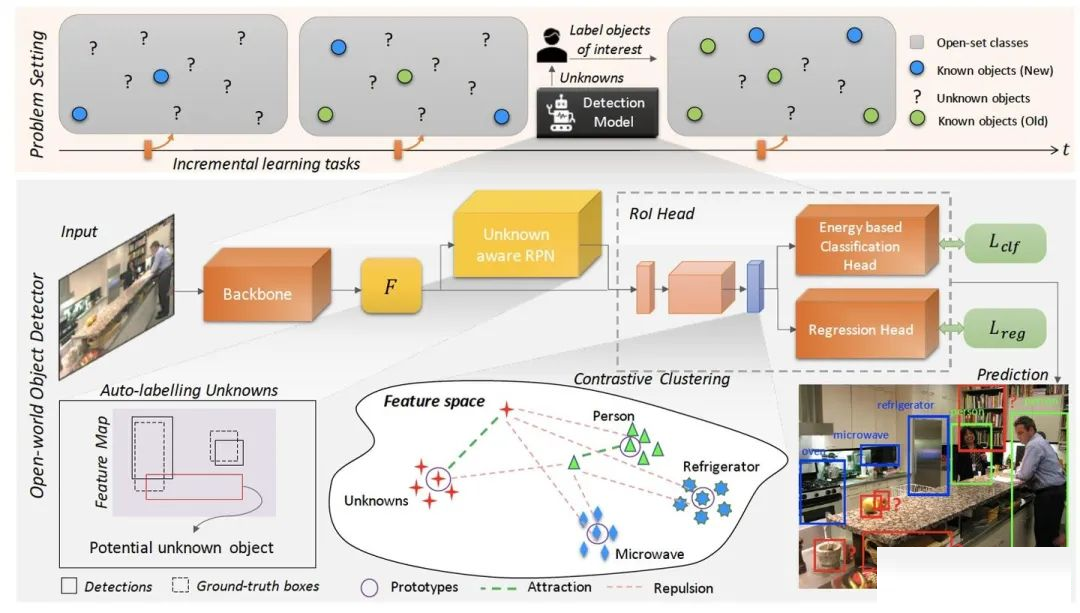
"OpenGAN: Open-Set Recognition via Open Data
Generation"

“Large-Scale Long-Tailed Recognition in an Open World“
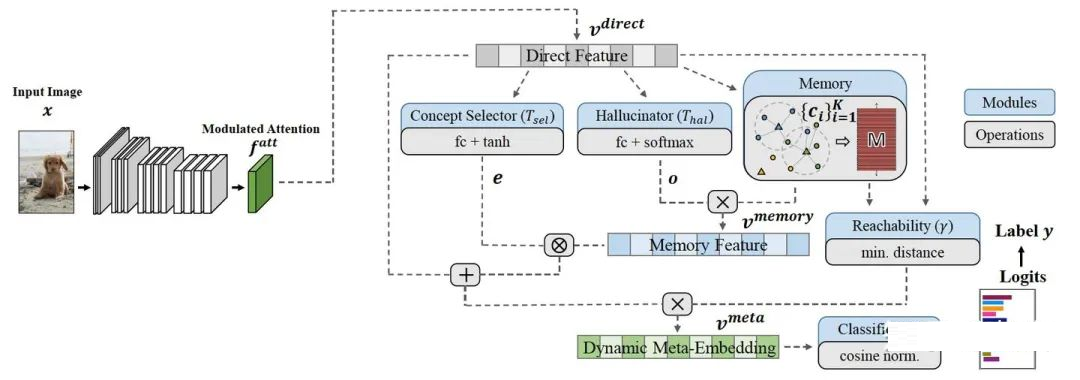
数据闭环的关键是数据,同时采用数据驱动的训练模型是基础。决定了整个自动驾驶迭代升级系统的走向是:
数据的模式(摄像头/激光雷达/雷达,无/导航/高清地图,姿态定位精度,时间同步标记);
数据驱动模型(模块/端到端);
模型的架构(AutoML);
模型训练的策略(数据选择)。
|