编辑推荐: |
本文于csdn,主要为TensorFlow是什么,TensorFlow特点、安装和使用。数据获取和输入等。
|
|
TensorFlow是什么?
TensorFlow是Google开源的第二代用于数字计算(numerical computation)的软件库。它是基于数据流图的处理框架,图中的节点表示数学运算(mathematical
operations),边表示运算节点之间的数据交互。TensorFlow从字面意义上来讲有两层含义,一个是Tensor,它代表的是节点之间传递的数据,通常这个数据是一个多维度矩阵(multidimensional
data arrays)或者一维向量;第二层意思Flow,指的是数据流,形象理解就是数据按照流的形式进入数据运算图的各个节点。
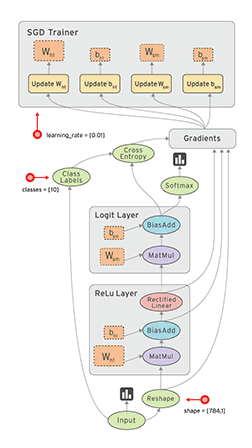
TensorFlow是一个非常灵活的框架,它能够运行在个人电脑或者服务器的单个或多个CPU和GPU上,甚至是移动设备上。TensorFlow最早是Google大脑团队为了研究机器学习和深度神经网络而开发的,但后来发现这个系统足够通用,能够支持更加广泛的应用。至于为什么谷歌要开源这个框架,它是这样回应的:
“If TensorFlow is so great, why open
source it rather than keep it proprietary? Theanswer
is simpler than you might think : We believe that
machine learning is akey ingredient to the innovative
products and technologies of the future . Research
in this area is global and growing fast , but lacks
standard tools. Bysharing what we believe to be one
of the best machine learning toolboxes in theworld
, we hope to create an open standard for exchanging
research ideas and putting machine learning in products.
Google engineers really do use Tensor Flowin user-facing
products and services, and our research group intends
to share TensorFlow implementations along side many
of our research publications.”
TensorFlow特点
1. 灵活(Deep Flexibility)
它不仅是可以用来做神经网络算法研究,也可以用来做普通的机器学习算法,甚至是只要你能够把计算表示成数据流图,都可以用TensorFlow。
2. 便携(True Portability)
这个工具可以部署在个人PC上,单CPU,多CPU,单GPU,多GPU,单机多GPU,多机多CPU,多机多GPU,Android手机上等,几乎涵盖各种场景的计算设备。
3. 研究和产品的桥梁(Connect Research andProduction)
在谷歌,研究科学家可以用TensorFlow研究新的算法,产品团队可以用它来训练实际的产品模型,更重要的是这样就更容易将研究成果转化到实际的产品。另外Google在白皮书上说道,几乎所有的产品都用到了TensorFlow,比如搜索排序,语音识别,谷歌相册,自然语言处理等。
4. 自动做微分运算(Auto-Differentiation)
机器学习中的很多算法都用到了梯度,使用TensorFlow,它将自动帮你求出梯度,只要你定义好目标函数,增加数据就好了。听上去很诱人,暂时不知道具体咋实现的。
5. 语言灵活(Language Options)
TensorFlow使用C++实现的,然后用Python封装,暂时只支持这两种语言,谷歌号召社区通过SWIG开发更多的语言接口来支持TensorFlow。
6. 最大化性能(Maximize Performance)
通过对线程,队列和异步计算的支持(first-class support),TensorFlow可以运行在各种硬件上,同时根据计算的需要,合理将运算分配到相应的设备,比如卷积就分配到GPU上。
TensorFlow安装
具体安装可以参看这个链接:
https://www.tensorflow.org /versions
/master/get_started/os_setup.html
源码可以从这个链接下载:
https://github.com /tensorflow/tensorflow
安装很简单,没有尝试GPU:
# Ubuntu/Linux 64-bit
$ sudo apt-get install python-pip python-dev
# Mac OS X
$ sudo easy_install pip |
Install TensorFlow:
# Ubuntu/Linux 64-bit, CPU only:
$ sudo pip install --upgrade https: // storage.googleapis.com
/tensorflow /linux/cpu /tensorflow-0.6.0- cp27
-none-linux_ x86_64 .whl |
# Ubuntu/Linux 64-bit, GPU enabled:
$ sudo pip install --upgrade https:// storage.googleapis.com /tensorflow/linux/gpu/ tensorflow-0.6.0 - cp27- none- linux_x 86_64.whl |
# Mac OS X, CPU only:
$ sudo easy_install --upgrade six
$ sudo pip install --upgrade https:// storage. googleapis .com / tensorflow /mac/ tensorflow- 0.6.0 - py2- none- any.whl |
安装完成后,输入如下命令,检测是否安装成功。
$ python
...
>>> import tensorflow as tf
>>> hello = tf.constant('Hello, TensorFlow!')
>>> sess = tf.Session()
>>> print(sess.run(hello))
Hello, TensorFlow!
>>> a = tf.constant(10)
>>> b = tf.constant(32)
>>> print(sess.run(a + b))
42
>>> |
如果报无法获取
CPU
信息错误,可以在上述代码中,修改添加如下几行:
NUM_CORES = 4 # Choose how many cores
to use.
sess = tf.Session(
config=tf.ConfigProto (inter_op_ parallelism_threads = NUM_CORES,
intra_op_ parallelism_ threads = NUM_CORES)) |
基础使用
在使用TensorFlow之前,有必要了解如下几个概念:
1. 计算是用图的形式表示的。
2. Sessions是执行的入口,类似于SparkContext。
3. 数据是用tensor表示的。
4. Variables用来表示可变状态,比如模型参数。
5. 使用feeds和fetches从运算节点输入和输出数据。
TensorFlow计算框架要求所有的计算都表示成图,节点在图中被称为运算op(operation简称)。一个运算可以获得零个或者多个Tensors,经过计算,可以产生零个或者多个Tensors。一个Tensor是一个多维数组,举个例子,可以把一批图像数据表示成一个4维浮点数组[batch,
height, width, channels]
计算图是通过Session提交,一个Session决定图中的运算该到那个设备上去计算,比如是选CPU还是CPU。运算op产生的结果在python中是一个numpy.ndarray数组对象,在C和C++中是tensorflow::Tensor实例。
构建一个计算图
构建图首先有构建运算op,op不一定要有输入,可以用Constant商量来构建一个运算节点,然后把它的结果输出到另外的运算中。如下是利用Constant生成一个简单的运算,并且以此构建了一个计算图。
import tensorflow
as tf
# 创建一个1X2的矩阵matrix1
matrix1 = tf.constant([[3., 3.]])
# 创建一个2X1的矩阵matrix2
matrix2 = tf.constant([[2.],[2.]])
# 创建matrix1和matrix2的乘积运算,返回矩阵product
product = tf.matmul(matrix1, matrix2)
|
上面这个图中包含三个运算,两个constant()运算,一个矩阵乘法运算matmul()。要获得最终矩阵乘积的结果,需要用session提交这个图。
Session提交计算图
# 创建session对象
sess = tf.Session()
# 通过Session中run()方法提交计算图
result = sess.run(product)
print(result)
# ==> [[ 12.]]
# 运行完关闭这个Session
sess.close()
|
当Session运行完成后,需要手动关闭,当然你也可以利用“with”关键字,运行完自动关闭。
with tf.Session() as sess:
result= sess.run([product])
print(result) |
在分布式运行环境下,一般不需要手动指出哪些运算该放到哪些机器上,比如CPU,GPU,但是框架提供了手动设置的功能。
with tf.Session() as sess:
withtf.device("/gpu:1"):
matrix1 = tf.constant([[3., 3.]])
matrix2 = tf.constant([[2.],[2.]])
product = tf.matmul(matrix1, matrix2)
...
|
目前支持这几种设备:
"/cpu:0": The CPU of your machine.
"/gpu:0": The GPU of your machine, if
you have one.
"/gpu:1": The second GPU of your machine,
etc.
交互式使用
TensorFlow提供了一个类似iPython的机制,通过创建InteractiveSeesion对象来实现。
# Enter an interactive
TensorFlow Session.
import tensorflow as tf
sess = tf.InteractiveSession()
x = tf.Variable([1.0, 2.0])
a = tf.constant([3.0, 3.0])
# Initialize 'x' using the run() method of itsinitializer
op.
x.initializer.run()
# Add an op to subtract 'a' from 'x'. Run it and
print the result
sub = tf.sub(x, a)
print(sub.eval())
# ==> [-2. -1.]
# Close the Session when we're done.
sess.close() |
变量(Variables)
变量维护整个执行图过程中间的状态,比如Hadoop中的计数器就是一个变量。具体在机器学习任务中,当我们训练一个深度神经网络的时候,每一层的节点权重就是用Variable来表示的,在训练过程中,权重会不断地更新。看如下示例:
# Create a
Variable, that will be initializedto the scalar
value 0.
state = tf.Variable(0,name="counter")
# Create an Op to add one to `state`.
one = tf.constant(1)
new_value = tf.add(state, one)
update = tf.assign(state, new_value)
# Variables must be initialized by running
an`init` Op after having
# launched the graph. We first have to add
the `init` Op to thegraph.
init_op = tf.initialize_all_variables()
# Launch the graph and run the ops.
with tf.Session() as sess:
# Runthe 'init' op
sess.run(init_op)
# Printthe initial value of 'state'
print(sess.run(state))
# Runthe op that updates 'state' and print
'state'.
for _in range(3):
sess.run(update)
print(sess.run(state))
# output:
# 0
# 1
# 2
# 3 |
assign()是赋值运算,实际的执行是在 run() 被执行的时候开始。
数据获取(Fetches)
获取一个op的运算结果,可以通过调用Session中的run()方法,同时可以获得多个结果,所有结果获取只需要执行一次run请求。
input1 = tf.constant(3.0)
input2 = tf.constant(2.0)
input3 = tf.constant(5.0)
intermed = tf.add(input2, input3)
mul = tf.mul(input1, intermed)
with tf.Session() as sess:
result= sess.run([mul, intermed])
print(result)
# output:
# [array([ 21.], dtype=float32), array([ 7.],dtype=float32)] |
数据输入(Feeds)
之前介绍的数据输入使用Constant,直接输入一个明确的常量数据,TensorFlow同时提供了一种“占位符(placeholder)”方式,用来表示一个数据,然后在调用run,通过参数传入批量输入数据进去后,具体获取数据,具体可以如下示例:
# 创建两个输入input1和input2,这时这两个数据里面什么都没有
# 可以理解为申明了两个输入变量。
input1 = tf.placeholder(tf.float32)
input2 = tf.placeholder(tf.float32)
output = tf.mul(input1, input2) >
with tf.Session() as sess:
print(sess.run([output], feed_dict={input1:[7.],
input2:[2.]}))
# output:
<# [array([ 14.], dtype=float32)] |
具体输入是feed_dict。
|